Even as data has come flooding in, there is so much we do not know about India’s Covid-19 epidemic. Eighteen months on, we have only a few clues about how the epidemic evolved in a landscape of huge inequalities. Which communities have been hit hardest? How do poverty, caste, and religion, affect your chances of dying from the disease?
Covid-19, unlike tuberculosis, is not primarily a killer of the poor. But, like TB, it spreads most easily in crowded housing. Factors such as malnutrition and poor access to healthcare make severe disease and death more likely.
At the same time, the ways Covid-19 data is gathered and reported tend to mask the toll on more marginalised communities. Access to testing and hospital care reflect privilege. Where these are available, more cases and deaths get recorded. Official manipulation of data can further obscure the impact on communities with limited power to make their stories heard.
Uncounted pandemic deaths
Thanks to the efforts of journalists, we now have a source of information beyond official Covid-19 statistics: death registration data. This adds a new and important tool to help us unravel the story of India’s epidemic. The data is neither complete nor always easy to interpret; but for some cities and states it allows us to credibly estimate pandemic “excess deaths” over and above what we expect from previous years’ data.
This data has already shifted our understanding of India’s epidemic. It tells us that in terms of mortality India has been hit very hard, worse than much of Europe or North America, and comparably to countries such as Iran, Brazil and South Africa. The data highlights the absurdity of government narratives about how India was spared.
Across India, the ratio of excess deaths to recorded Covid-19 deaths, sometimes termed the “undercount factor”, could be as high as 9 or 10. But there are striking variations. In some states – Kerala, Maharashtra, Punjab and Himachal Pradesh, for example – this factor is between 3 and 5. In Bihar, Madhya Pradesh and Andhra Pradesh, it rises to over 20.
We don’t really understand why the official Covid-19 toll has captured such a small fraction of pandemic deaths. No doubt differences in development and infrastructure explain some of the variation. Deliberate manipulation plays a part too. There could be other factors in play. At least there is now a clear question to be asked: why have so many deaths gone uncounted?
As coronavirus devastates India, deaths go undercounted: https://t.co/KfGfQgjN8z via @nytimes
— Darshan Patel (@drshnpatel) April 24, 2021
Mumbai’s excess deaths
Against this backdrop, it is interesting to look again at Mumbai. During 2020, the city saw 11,116 official Covid-19 deaths, but roughly 22,000 more death registrations than expected, giving an undercount factor of 2. Allowing for some disruption to death registration, this could rise to 2.5.
The undercount factor in Mumbai is low by national standards. But one thing we know well is that data from Mumbai as a whole could hide very different stories in the slums and nonslum areas.
During June-July 2020 the city corporation, in partnership with the Tata Institute of Fundamental Research, surveyed residents of three wards for antibodies to the virus that causes Covid-19. Thanks to their crucial decision to sample slum and nonslum residents separately, we learned that the disease had swept rapidly through the city’s slums, but simmered more slowly in nonslum areas.
At the time of the survey, the infection rate in the slums, at around 56%, was more than three times that in nonslum areas. But only a tiny fraction – less than 1% – of slum infections had been captured in testing. The slum surge was largely hidden in case data.
Moreover, recorded Covid-19 deaths from the slums were surprisingly low. According to analysis of the serosurvey, only around 0.076% of those infected in the slums had died of the disease. For nonslum areas this figure was more than three times as high, at 0.263%. These are estimates of the so-called infection fatality rate, or IFR, of Covid-19 in the slums and nonslum areas based on the official death count.
Reasons for suspicion
Given that the risk of severe disease rises rapidly with age, big variations in IFR need not be surprising. One line of argument goes: “Yes, disease spread rapidly in the slums. But there was a silver lining: migration and low life expectancy have led to a young slum population, and consequently fewer Covid-19 deaths.” This sometimes continues with: “The slums are lucky: they got their epidemic out of the way early with relatively few deaths”.
Even if there is a grain of truth in some of this, we should be suspicious of any narrative which finds a silver lining in low life expectancy. It is likely that the factors that decrease life expectancy, including chronic untreated health conditions, also increase the risk of a Covid-19 infection proving severe or fatal.
Moreover, monthly excess deaths data immediately calls into question the story of low slum mortality.
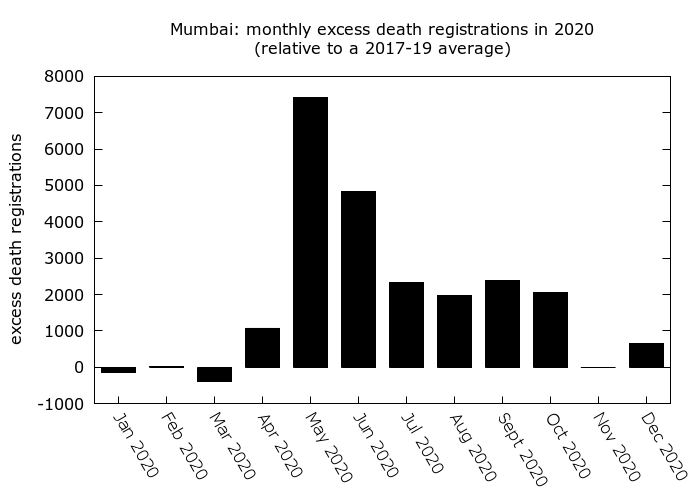
What stands out is the huge rise in death registrations during May and June, around the time of the slum surge. About 60% of total excess registrations for the year had occurred by the end of June, and almost 70% by the end of July. This is despite the fact that the bulk of Covid-19 cases for 2020 were added later in the year during a major surge in housing societies.
The early part of the year also saw the greatest mismatch between excess deaths and official Covid-19 deaths: the undercount factor was almost 3 up to the end of June, even after a reconciliation in June that added a large fraction of the city’s official death toll. But it fell to around 1.5 between August and December.
The timing of the surge in mortality, and the high early undercount factor which later fell, hint that many of the city’s excess deaths may have occurred in the slums and not been recorded as Covid-19 deaths.
What was the death toll in slums and nonslum areas?
With some calculations we can try to reconstruct slum and nonslum excess deaths. A plausible scenario gives similar levels of excess mortality in the slums and nonslum areas during 2020, with around two excess deaths per thousand population in both halves of the city. The greater spread of disease in the slums effectively cancelled out any benefits of a youthful population.
In this scenario, using excess deaths rather than official Covid-19 deaths to estimate IFR, we find IFR in the slums to be around 0.26%, and in nonslum areas around 0.47%. IFR in nonslum areas was likely higher than in the slums; but the difference is not as dramatic as the recorded death toll suggests.
These estimates give an undercount factor of around 3.2 in the slums, and 1.7 in nonslum areas. This would mean that roughly 60% of pandemic excess deaths in nonslum areas were officially recorded as Covid-19 deaths; but only 30% in the slums.
If most of the city’s excess deaths were from Covid-19, then many more went unrecorded in the slums compared to nonslum areas. Was this about a lack of testing in the slums? Or because many slum dwellers could not find hospital beds? Are there fundamental flaws in the process of recording Covid-19 deaths which lead to these disparities? These are unanswered questions.
Tweet does not exist
Inequality, marginalisation and unrecorded pandemic deaths
The broad point here is not about Mumbai. Mumbai’s data simply highlights the risk of underestimating the impacts of the pandemic on the most marginalised communities. Narratives built on incomplete data such as “low mortality in the slums” can become widely believed, whether or not they are true.
Mumbai’s case data indicates that both slums and nonslum areas were hit badly again in 2021. But were deaths in the slums much reduced this time, thanks to the protective effects of prior infection? This is plausible, but without adequate data, we shouldn’t rush to assume so. Death registration data for this year is incomplete, but suggests that excess deaths were again high – and again more than double official Covid-19 deaths. It is unclear where these deaths occurred.
Ultimately, to understand where and why so many of India’s pandemic deaths have gone unrecorded, we need to push for more information. More granular death registration data and mortality surveys would help make sense of the story. The process of understanding begins with asking the right questions.
Murad Banaji is a mathematician at Middlesex University, London.
Detailed analysis and references to the data that inform the claims here about Mumbai can be found in a preprint on Mumbai’s Covid-19 epidemic by this author.