Artificial intelligence has immense potential to enhance human capabilities and drive growth in several industries. It is projected to greatly improve governance, healthcare, and education outcomes.
However, this potential may not be realised if the building blocks of AI remain concentrated in the hands of a few dominant companies or the countries in which they are located.
The priorities for AI adoption in India can be quite different. Vijay Kelkar and Ajay Shah propose that the toughest challenges for a state – such as the tax system – involve processes that feature a high number of transactions, the need for discretion, high stakes for individuals, and some degree of secrecy.
AI adoption could reduce the complexity of such challenges on some of these dimensions, such as the transaction volume and discretion. This makes it easier to overcome state capacity limitations and deliver better governance and public services.
On the other hand, widespread AI adoption could also negatively affect the availability of low-skilled jobs upon which a large part of India’s labour force depends. Thus, the opportunities and challenges for India might be significantly different from those of developed countries.
Technology and geopolitics are becoming increasingly intertwined. Many countries have identified critical and emerging technologies that are essential for national security and economic growth. AI features on the lists of all countries that have made such declarations, including the United States, the United Kingdom, the European Union, Australia, and Japan. These technologies are not just an area of focus but also of strategic interest.
For instance, US policymakers are operationalising the idea of “a small yard with a high fence” for critical technologies, with the aim of keeping the chokepoints for foundational technologies under US control. From their longstanding approach of staying a few generations ahead of rivals in critical technologies, the American focus is now to “maintain as large of a lead as possible”.
Given these considerations, for India to pursue its national interests, it will have to find ways to maintain strategic autonomy with respect to this critical technology.
Inputs
The development of AI systems requires different inputs: data, computation, models, and applications. These inputs can be visualised as layers, with data and computation contributing to the model, which, in turn, supports the applications.
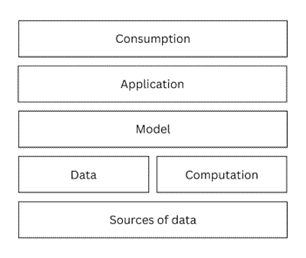
Companies involved in developing AI models or applications face entry barriers at each of these stages. It is not uncommon for a single company to control multiple stages through vertical integration.
For instance, Google exemplifies a high level of integration across the different stages of the supply chain. Its operations range from developing and training its own AI models on proprietary computing infrastructure using vast amounts of data, including proprietary data. It also offers cloud services and has integrated its AI systems into various applications for both web and Android users.
Given this context, if India is to build a thriving domestic industry with local companies that can compete with global giants, AI governance will need to ensure unfettered access to the different stages of the supply chain. A number of primary considerations are necessary for each stage of the supply chain.
Data
Models trained on large, diverse, and high-quality data sets tend to perform better. Studies estimate that for training large language models, high-quality data from sources such as books, academic papers, news articles, and Wikipedia are likely to be exhausted by 2027.
Thus, access to proprietary data can be a key differentiator for training AI models. This includes data such as the extensive code repositories available on GitHub, the sorted and categorised web index compiled by search engines from their web crawling activities or the audio and video recordings from video conferencing applications such as Zoom.
Big tech platforms tend to be monopolies or duopolies due to the network effects. This market consolidation is evident across various platforms, ranging from search engines and social media to ride-sharing and food-delivery services.
The scale and insight into user behavior from access to proprietary data help these companies innovate better than competitors. When developing AI applications, access to proprietary data gives these companies an edge. It also helps them integrate the AI applications into their existing offerings, thereby further consolidating market power.
Research also shows that issues with fairness exist in AI use cases in medical diagnoses, gender classification, recidivism prediction and other areas where certain groups are underrepresented in the training data. This can lead to higher error rates and biased outcomes for minorities, women, the elderly, and vulnerable populations.
Given India’s incredible diversity, the government could play an important role in creating publicly accessible open datasets that are representative of the population. Such datasets can have significant positive externalities for both research and commercial applications.
Bhashini is one such initiative under the Indian government that attempts to capture the diversity of Indian languages and provide open-source databases and tools for real-time translation.
Computation
AI training and usage typically relies on cloud computing services for computational infrastructure, a preferred choice for developers and users over owning the hardware. Cloud service providers often offer substantial discounts on computing resources for AI research and development, aiming to secure a strong market position as the sector expands.
Despite its advantages, this model raises concerns. Due to the economies of scale, major Cloud service providers such as Amazon Web Services, Microsoft Azure, and Google Cloud dominate the market,collectively holding a 65% share globally. While this market concentration is not a cause for concern, the leading cloud service providers also compete in other stages of the AI supply chain, including developing models and applications.
Ensuring that vertical integration with cloud computing services does not hinder competition in other parts of the supply chain is crucial. Competition regulators – including in India – must be vigilant about anti-competitive practices such as restrictive contracts, high costs for switching services, or unfair pricing strategies.
Further upstream, NVIDIA, a chip manufacturer, holds more than 90% of the market share for graphics processing unit, the semiconductor chips essential for AI-related tasks. This market dominance stems from NVIDIA’s early entry into the market and the widespread adoption of its proprietary computing platform, CUDA. While intense competition is likely to create alternatives in the long term, this remains a geopolitical risk that needs to be mitigated.
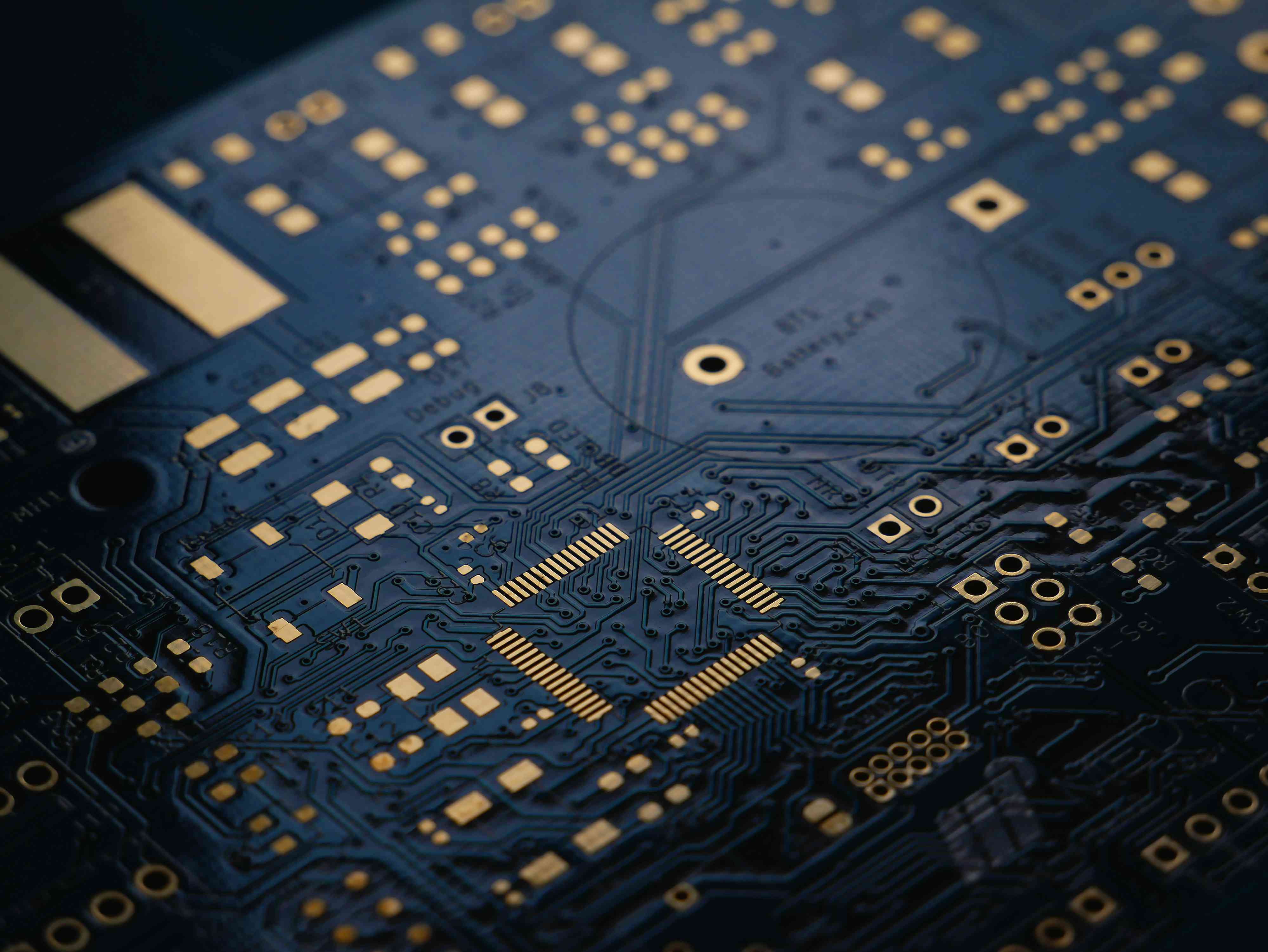
Models
A report from Bain & Company highlights that India is a leading AI talent hub, accounting for 16% of the global AI workforce, and positioning it among the top three in the world.
However, there’s a notable scarcity of top-tier AI researchers in India, those engaged in generating intellectual property or in designing and training AI algorithms. Research by MacroPolo, a US think tank, indicates that over 80% of India’s premier AI researchers relocate abroad.
These trends suggest that due to the shortfall in specialised skills, coupled with the barriers in the data and computation stages, Indian companies – which rely heavily on Indian talent – would find it challenging to create world class models. However, they will be able to compete effectively in other engineering skill-based tasks such as developing applications based on AI models.
Additionally, developing cutting-edge AI models demands substantial data, computing power, and technical expertise, and the industry can mobilise these resources better than academia.
This trend is underscored by the Stanford AI Index report, which reveals that in 2022, industry entities produced thirty-two significant machine learning models, in contrast to academia, which contributed only three. Thus, government interventions to build AI capabilities should consider both the industry and academia as key contributors to innovation.
Application
Finally, addressing concerns in other stages of the AI ecosystem will pave the way for a competitive market in the application stage. However, a framework to better manage risks associated with AI systems is required to ensure they are built responsibly. Alignment with AI risk management frameworks, such as the one developed by the US’s National Institute of Standards and Technology, can help systematically assess and mitigate risks across the development life cycle.
As global efforts are underway to better understand and govern AI, the Indian state needs to consider its unique conditions and the current geopolitical climate to take measures to ensure access to the technology and the benefits it has to offer.
This article and the discussion document on which it is based focus on AI governance from a national interest perspective, highlighting some of the primary concerns at each stage of the AI supply chain. Recognising these concerns is crucial, as they lay the groundwork for shaping targeted policy measures tailored to each stage.
Bharath Reddy is a researcher in the High-Tech Geopolitics programme at the Takshashila Institution. He is a co-author with Nitin Pai, Satya Shoova Sahu, Rijesh Panicker and Sridhar Krishna on Takshashila’s discussion document on AI Governance.
The article was first published in India in Transition, a publication of the Center for the Advanced Study of India, University of Pennsylvania.