Is someone named Rhea Ahluwalia more likely to be affluent than someone named Panna Lal?
If your instinctive answer was “Yes”, you would – ceteris paribus – be right. Indian names, both first and last, contain enormous signalling value. They can be used by marketers, analysts and entrepreneurs to assess the affluence, age, ethnicity and gender distributions of their audience.
Methodology
At Loki.ai, we analysed more than 100 million names from publicly available sources to develop algorithms that predict audience demographics and affluence based on the names of individual users.
To do this, we cleaned and analysed data from electoral rolls, CBSE results and matrimonial sites. We then used the cleaned data to train our models for various tasks. We only scraped data from public sources and ensured that we were not in violation of terms of service at the time of scraping.
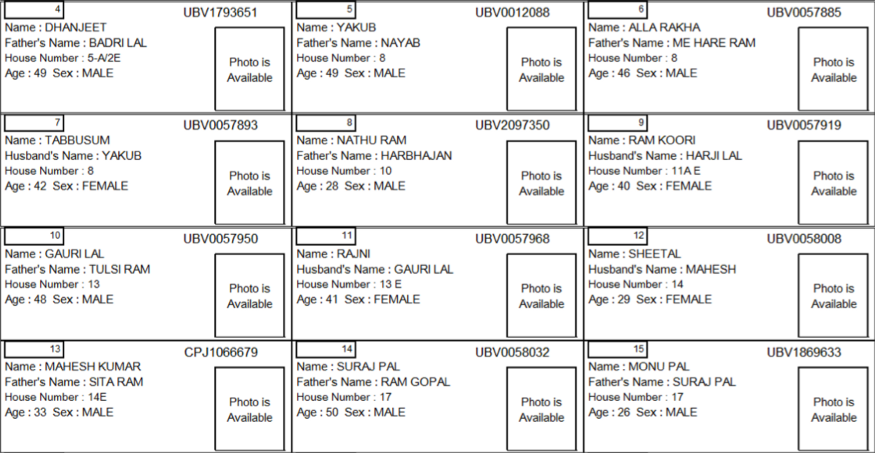
Age prediction
Intuitively, most Indians recognise that names such as Shubham and Rishabh are younger and more modern names, while names like Om and Shashi are older names.
We quantified this by creating an age histogram for individual names. For instance, a cursory look at the graph below shows that the name Abhishek skews young.
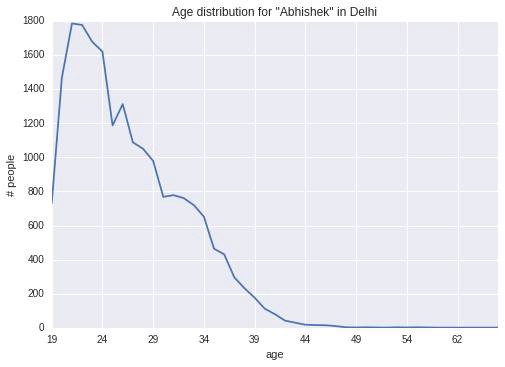
We used the skew of these histograms to create an age-index for each first name in India. The lower the age-index for a name, the younger a name is likely to be. The graph below shows the age-index for selected names.
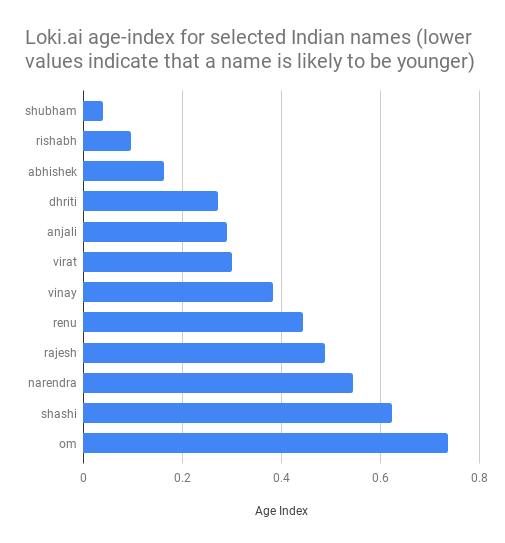
There is one glaring problem with this index – it is based only on the names of adults and does not reflect data for those aged 18 or less. But it is fairly accurate for adult users in India.
Affluence estimation
We also overlaid electoral roll data with data scraped from property sites to discover correlations between where different communities lived and where property and rental prices were the highest.
For instance, property prices in Bankner in Delhi are far lower than property prices in areas like Rajouri Gardens and Rohini. We could draw correlations between these prices and the distributions of different communities in these areas. The image below, for instance, lists the nine most common surnames in selected communities in Delhi.
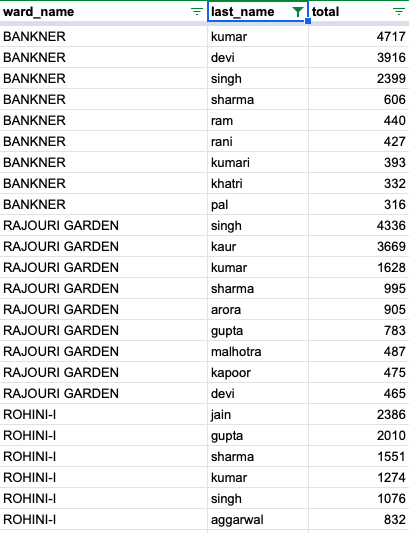
We did a similar analysis for first names. Additionally, we also looked at educational achievements in CBSE results and also used those as a proxy for affluence. This is a questionable assumption but was one that we believed improved our model.
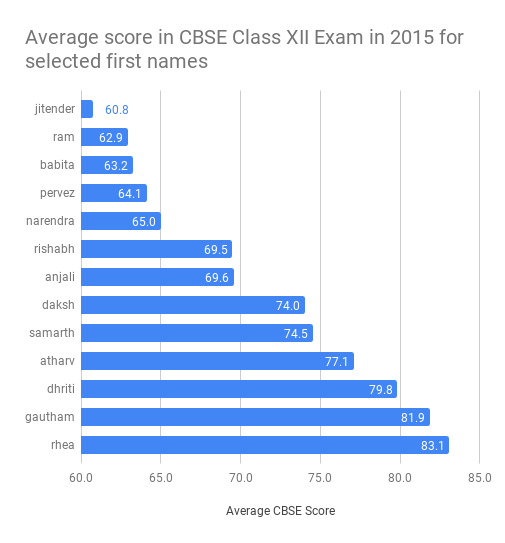
Using a combination of these factors, we developed an algorithm that predicts an affluence index for each name – based on both and first name and the last name.
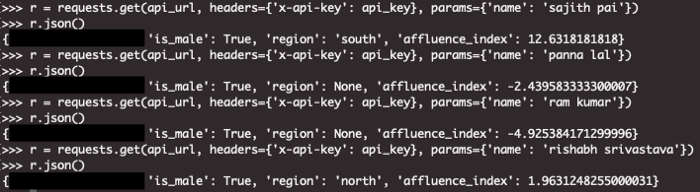
An affluence index greater than zero is considered more affluent than the average internet user in India, while less than zero is considered less affluent than the average internet user in India.
The name Sajith Pai, for instance, has an extraordinarily high affluence index of 12.6. My name, Rishabh Srivastava, has a higher than average affluence index of 2.0. The names Panna Lal and Ram Kumar has affluence indices of -2.4 and -4.9, respectively.
It’s important to note that the algorithm does not have a use case for predicting affluence at an individual level – especially in the field of recruiting or lending. As a case in point, the name Shailendra Singh has an affluence index of -3.2. However, the Managing Director of Sequoia Capital India is also named Shailendra Singh – and is clearly unlikely to be less affluent than the average Indian.
The algorithm is, however, fairly good at predicting the average affluence of a large group of users.
Ethnicity prediction
Rishabh Srivastava, my name, is highly likely to be that of a North Indian, while a name like Ronojoy Sen is highly likely to be that of someone who is ethnically Bengali.
We can train a model to learn these patterns by feeding it labelled data. To this end, we scraped the names of people who reacted to stories in regional languages on social media.
Using this, we were able to build an accurate model that can predict someone’s ethnicity using their last names and first names. For instance, Simran Ahluwalia is likely to be Punjabi, Abhishek Vaidya is likely to be Gujarati, Megha Bhattacharya is likely to be Bengali, and Inian Parameshwaran is likely to be Tamil.
The model isn’t perfect. A name such as Shiv Kumar – which can be both a North Indian name and a Tamil name – will result in weak predictions. But it is fairly accurate for most cases.
Gender estimation
Cleaning the electoral rolls also gave us heaps of labelled gender data to train on, which we duly did. It was fascinating to see that there are instances where the first name is less indicative of a users’ gender than the last name. For example, Harmanpreet Kaur is highly likely to be female, while Harmanpreet Singh is highly likely to be male.
Applications
We built these tools mostly as a fun learning project, but have since learnt that they have a number of commercially useful applications.
Using names can help brands measure the demographics and affluence of their audience, as well as that of the publishers they advertise with. Similarly, publishers can use names to find out what kind of stories attract what kind of audiences.
For instance, when we did an analysis of reactions on Facebook pages from May to July 2017, we found that light content pages like BuzzFeed India and Vagabomb had the most affluent audiences, while hard news pages like NDTV and Zee News attracted audiences with relatively less affluence.
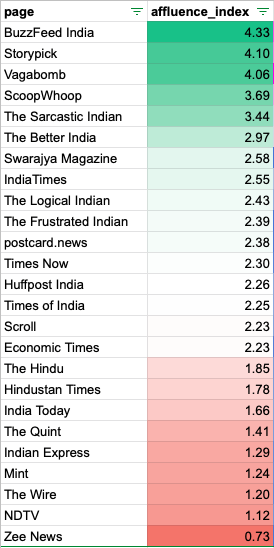
On a similar note, Hindustan Times stories that attracted disproportionately more women were about soft content. Stories that attracted disproportionately more men were about cricket and politics.
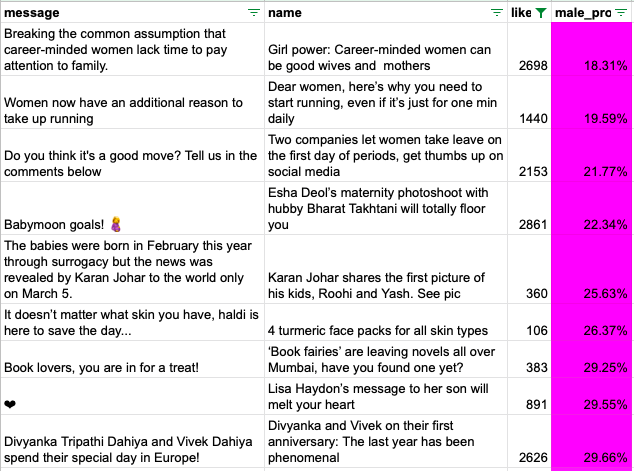
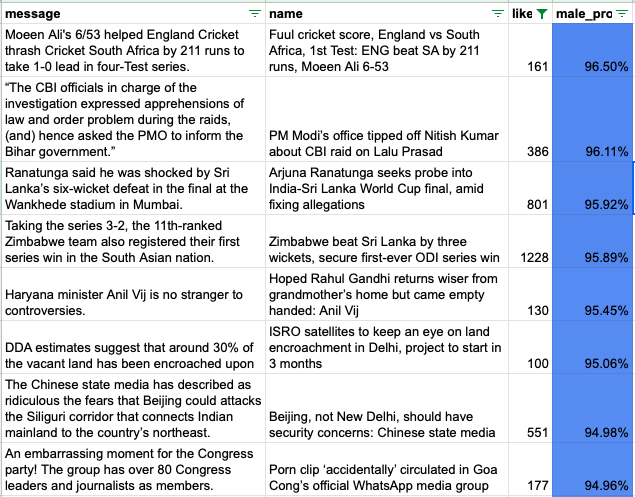
Efficacy of customer acquisition strategies
Using online social channels for customer acquisition in India is fraught with danger. It’s hard for one to control the kind of audience they are acquiring. By the time the expected lifetime value of customers acquired from a campaign becomes clear, a lot of marketing dollars have already been spent.
For example, Mint did a paid partnership with Honeywell India and used Facebook’s Boost Post functionality to ensure that the message went out to a larger audience.
But as the comments below indicate – doing so did not attract an audience that was likely to buy Honeywell’s products. This post had an average affluence index of -2.3, much lower than Mint’s average affluence index of 1.24.
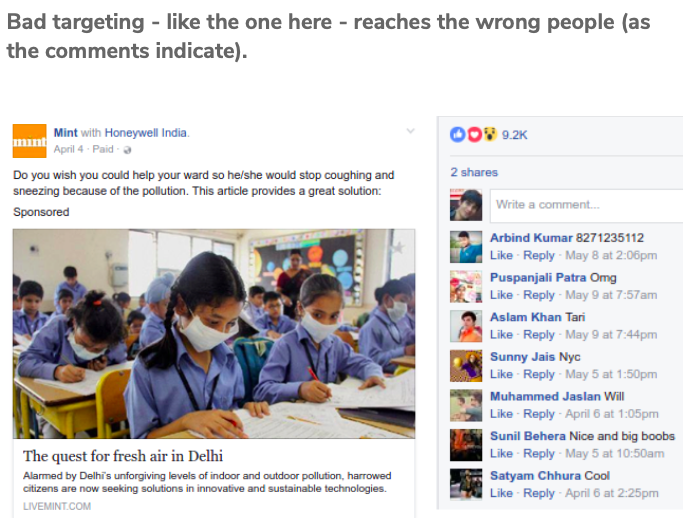
Calculating the affluence index of users acquired from campaigns in real time can help brand stop a campaign that acquires the wrong kinds of users before too many marketing dollars have been spent.
Name analytics can also be used by e-commerce and content websites to target specific demographics with specific campaigns. For example, Marathi users can be targeted with campaigns linked to Ganesh Chaturthi. Similarly, Bengali users can be targeted with campaigns linked to Durga Puja.
Rishabh Srivastava is a data scientist and founder of Loki.ai.
This article first appeared on the writer’s Medium page.