The nationwide lockdown in India has caused a humanitarian crisis but failed to stem the rise of Covid-19 cases. Nevertheless, on May 22, the government released the results of a number of models, all suggesting that the lockdown had saved thousands of lives.
The most dramatic study was by the Boston Consulting Group, which used a so-called SEIR model to claim that the lockdown had saved between 120,000 to 200,000 lives. Some independent scientists working on a model called INDSCI-SIM also chimed into the debate a few days later. Although they urged the government to release more details about its estimates, they announced that their own model suggested that the lockdown had averted between 8,000 to 32,000 deaths.
It is important to examine these claims since they have a bearing not only on how we evaluate the lockdown but also on future policy decisions.
We must first understand what simple epidemiological models tell us about deaths averted by the lockdown. This will lead us to more realistic questions about the lockdown, which cannot be answered by simple models, and also to some of the broader concerns about the use of mathematical models to make such claims.
What do simple epidemiological models say about the effect of the Indian lockdown?
Both SEIR models and INDSCI-SIM are simple epidemiological models of a kind that are known as “compartmental models”. Compartmental models divide the population into various groups: a group of susceptible individuals, another group of those exposed but not yet infectious, a third group of those who are mildly symptomatic, a fourth group of hospitalised individuals and other categories.
Deceased individuals can themselves constitute a compartment, and the fatality rate may depend on the number of hospitalisations and other parameters. The models then posit a simple set of differential equations to describe how the occupancy of compartments changes with time.
Such models, when applied to the Covid-19 pandemic, uniformly predict that a lockdown of the kind witnessed in India – which is imposed very early in the epidemic but fails to quash it – will only delay deaths but not avert them.
More precisely, the prediction for the final numbers of “deaths averted” is much smaller than other systematic errors, which are always present because models are forced to simplify reality.
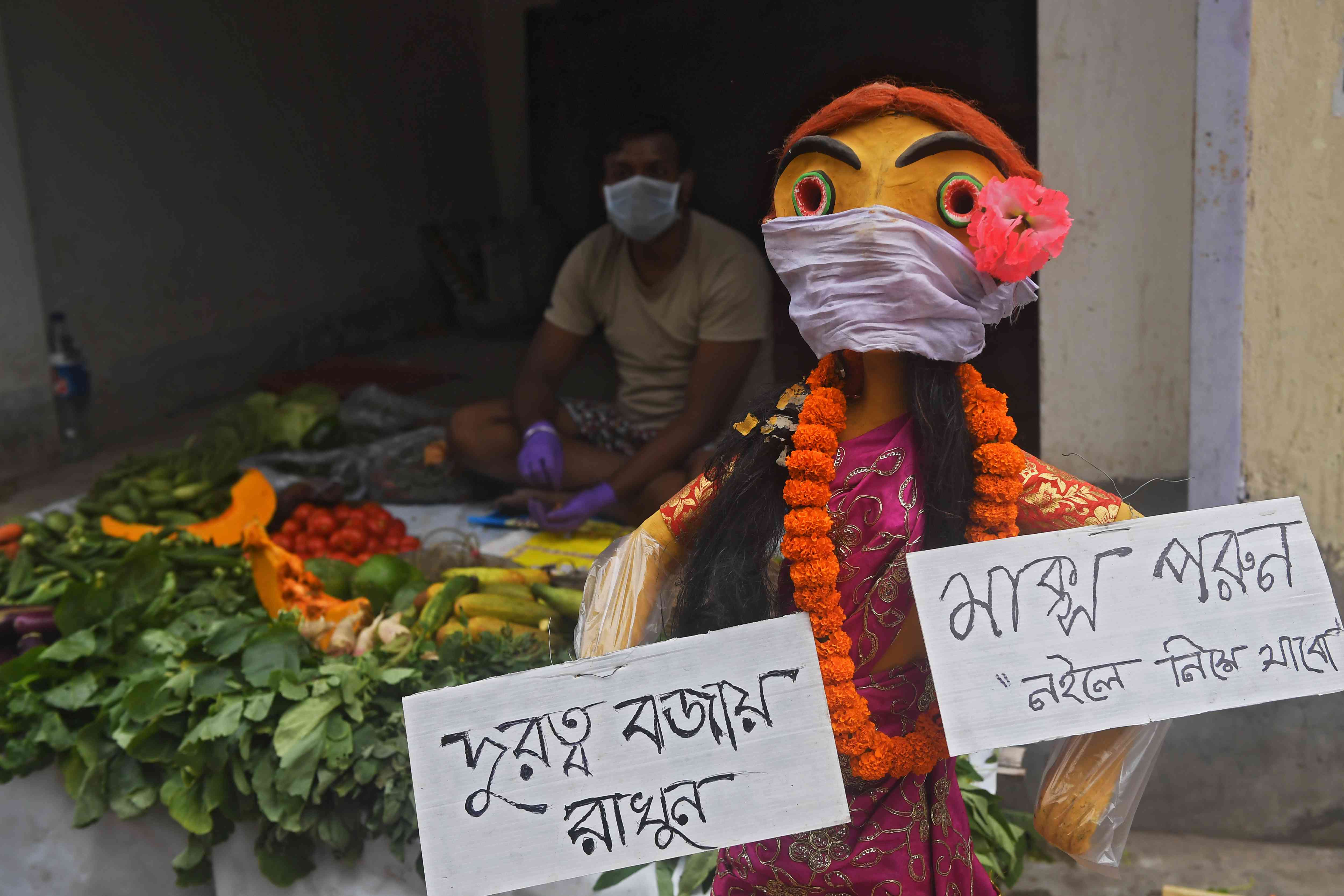
When a lockdown is imposed very early in the epidemic, most of the population is susceptible and the occupancy of other compartments is small relative to the population. In India, the official figure for the fraction of infected individuals when the lockdown started on March 25 was only four in ten million.
Therefore when the lockdown is released, the fractional occupancy of the compartments is necessarily similar to what it was at the start: with most people susceptible and only small fractions of the population in other compartments. So the subsequent trajectory of the model, including the number of fatalities, is similar to the trajectory without a lockdown, albeit with a time delay.
The post-lockdown trajectory may differ from the pre-lockdown trajectory, if one accounts for the fact that people themselves take precautions and alter their behaviour during an epidemic. But this effect is independent of the lockdown. This is a simple and well-known result but, for those interested in more details, it is described more formally in this accompanying note.
An analogy from a playground might help to make this clear. Consider two balls that are released from the top of a slide, and come to a stop after rolling down the slide and some distance along the ground. If one puts out one’s hand and slows down one ball near the top of the slide, the other ball will race ahead and seemingly build up a large lead. But when the first ball is released, its final resting position will be close to the second one.
This analogy captures another aspect of the lockdown. The first ball will come to rest at a slightly smaller distance than the second one, because by slowing it down, one made it lose some of its kinetic energy. But if this slowing-down was done very close to the top of the slide, then the final impact will be very small.
If models suggest that lockdowns delay and do not avert, where do the numbers for ‘deaths averted’ come from?
Surprisingly, none of the groups whose studies were cited in the public announcements about “deaths averted” have, to my knowledge, as yet published complete details about their calculations.
But by looking at various graphs and tables presented with these results, the methodology of such studies can be guessed. The studies appear to have taken data for fatalities in India, extrapolated the early rate of growth to the middle of May using an assumption of exponential growth (which is common to all models) and compared that number with the recorded fatalities on the same date.
Exponential functions, with a small difference in the exponent, can diverge widely when followed over a long period. Since the early data is noisy and flawed, one can fit various exponentials to it. This is what allows one group to get 8,000 for this difference and another group to get 200,000 for a similar quantity.
But this number is meaningless and should not be equated with the “deaths averted” due to the lockdown. Part of the difference between the early and late rates of growth can be attributed to the physical-distancing enforced by the lockdown, and the models themselves suggest that this is a transient effect. Part of the difference can also be attributed to simple precautions that people themselves have taken, including wearing masks and maintaining distance where possible. This effect cannot be credited to the lockdown.
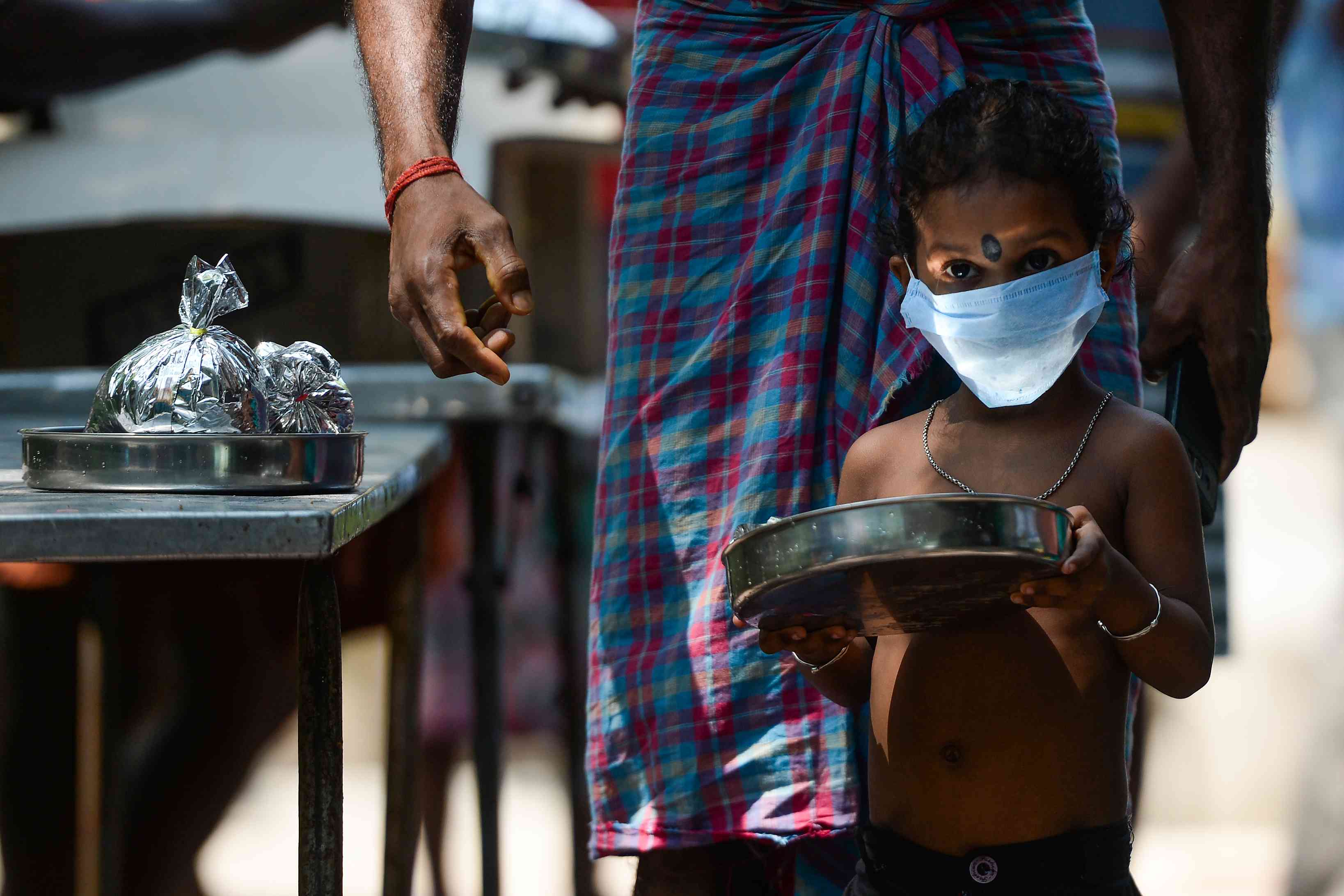
Does this mean that lockdowns have no long-term impact on fatalities in real life?
No. In real life, lockdowns can have a positive or a negative impact on fatalities that cannot be captured by simple models. An obvious example is the lockdown in Hubei in China, which brought down the level of infections so significantly that the epidemic there has since been kept in control through testing and tracing. Lockdowns in other countries have had similar effects, although this is evidently not the case in India.
Lockdowns may also give time for the healthcare system to ramp up its capacity, including its ability to test and quarantine individuals. But it is difficult to quantify the progress that India has made on this front.
Lockdowns also have several negative impacts. In India, the single-most important effect of the lockdown is the humanitarian crisis that it has caused. The lockdown has also reduced access to healthcare. Even a small increase in the fatality rate of a disease like tuberculosis, which already claims about 450,000 lives a year, could overwhelm any small gains made by the lockdown in averting fatalities from Covid-19.
Moreover, the Indian lockdown has engendered economic insecurity. As a result, it has become harder for people to maintain physical-distancing in the long run. Some measures, such as staggering the opening-hours of shops, which could have helped moderate the spread of the epidemic in the long-run are now difficult to implement since they impose an additional economic cost on people.
Why not improve the models to include all these real-world effects?
This is theoretically possible but the available data is so limited that this exercise often ends up simply reflecting the ideological biases of the modellers.
For instance, the INDSCI-SIM model includes a parameter that accounts for a steady increase in testing and quarantining capacity. The effect of this parameter is to cause an exponential decay in the rate at which infected individuals transmit the infection.
But we have no way of estimating the value of this parameter in India, or even of knowing whether this effect is particularly significant at the moment given India’s low rates of testing and possibly high proportion of undetected cases. And for this reason, this parameter appears to have played no meaningful role in the modellers’ estimate of “deaths averted”, which appears to have used the same procedure of naively extrapolating an early exponential.
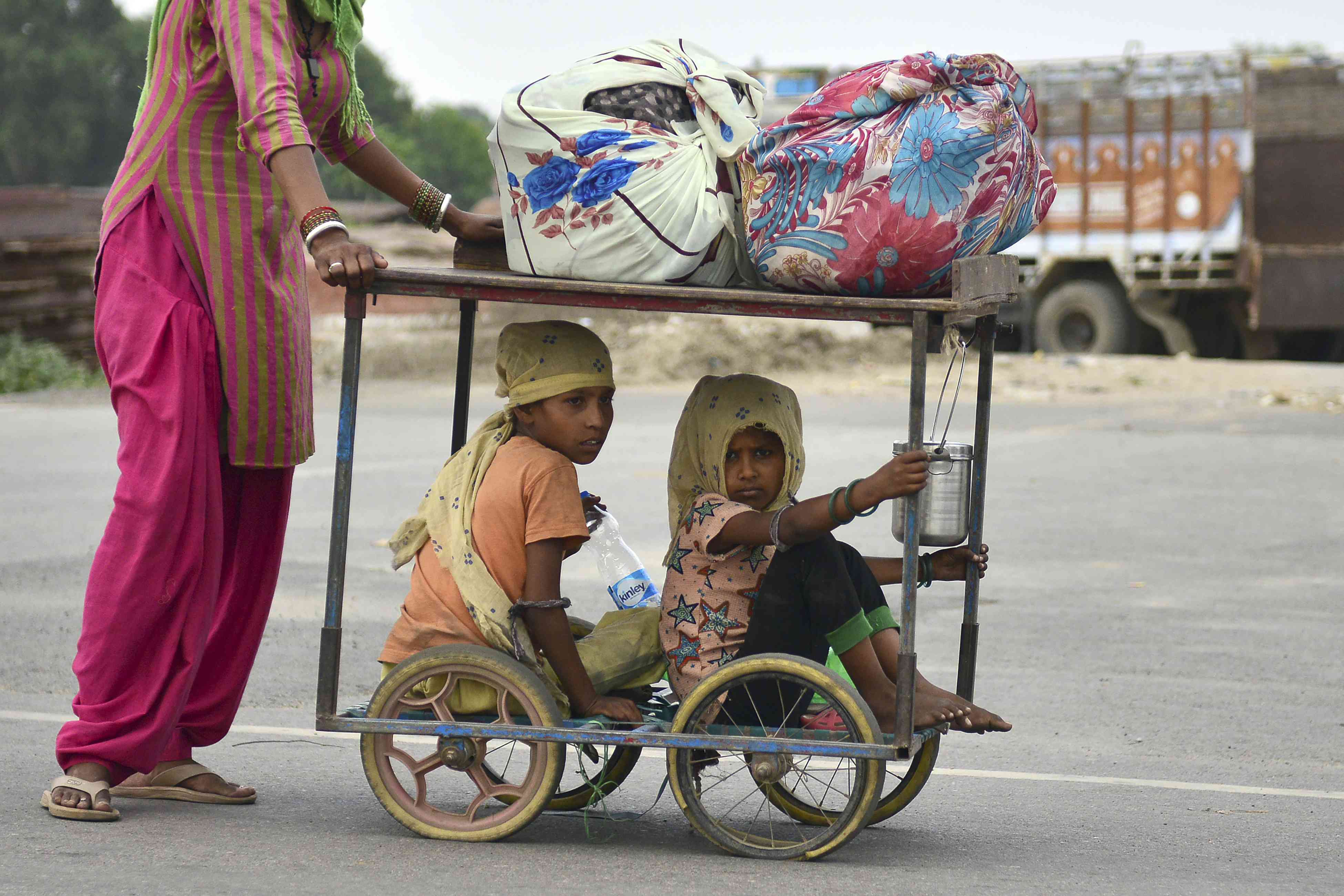
Nevertheless, in a note released on April 18, the modellers did use this parameter to provide a theoretical justification for the lockdown. They argued that with appropriate hypothetical values for this parameter, a lockdown could avert deaths in the long run. But, given the uncertainty in the value of this parameter, this analysis only reflects a bias and is not a reliable scientific recommendation in favour of the lockdown as policy.
It is easy to come up with models that make the opposite prediction. For instance, one could introduce a parameter into the model that links increasing levels of economic insecurity to increasing rates of infection-transmission, as described above. By setting this parameter to an appropriate value, and noting that a lockdown increases economic insecurity, one could argue that a lockdown will increase the final toll of the epidemic and even calculate some hypothetical numbers for this increase.
But these numbers would be equally unscientific, since we do not have enough data to accurately quantify the relationship between economic insecurity and increased transmission rates.
What is the conclusion: has the Indian lockdown averted deaths or worsened the pandemic?
The simple answer is that we just cannot estimate this quantitatively. There are so many intangibles associated with this question that only a qualitative analysis is possible.
My own opinion is that the humanitarian crisis, the impact of the lockdown on healthcare for other diseases, and economic difficulties that have made physical-distancing difficult are all more important than the small improvements, made during the lockdown, in the capacity of the healthcare system to address Covid-19. So I believe that the lockdown has exacerbated the challenge of the pandemic, and will probably worsen its final toll.
But I cannot quantify this expectation. And, most importantly, the fact that I know how to model differential equations on a computer gives me no special insight into evaluating the lockdown. Any other concerned citizen could look at the publicly available data, think about the question, and come to her own equally-valid conclusions.
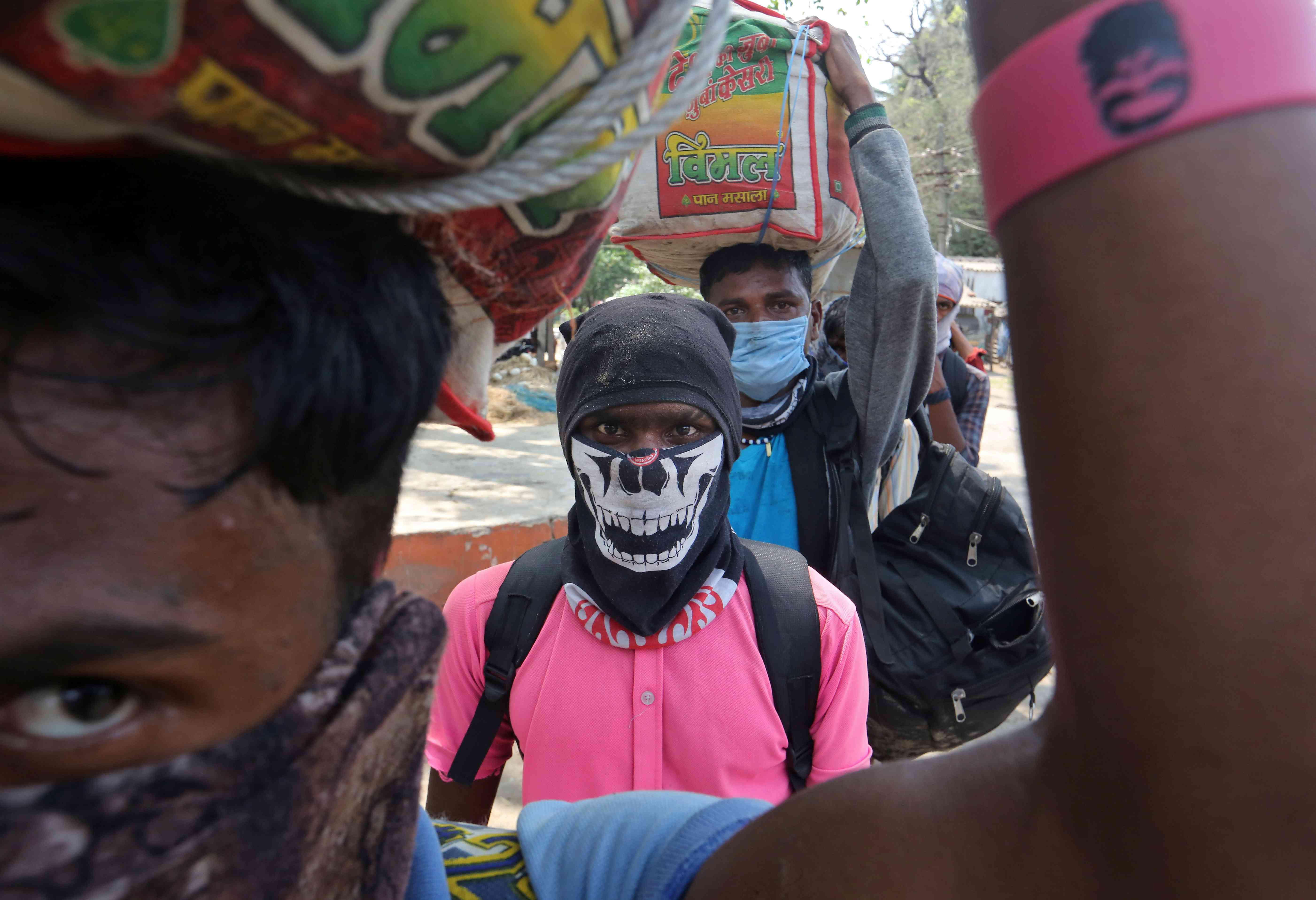
If models cannot say much about the effect of the Indian lockdown on deaths, what motivates these studies?
I would like to separate the independent modellers from the ambit of this answer since I am sure they were acting in good faith and perhaps inadvertently fell into a trap set by the government.
But, as for the government-backed studies themselves, the answer is obvious. There is increasing concern in the country that the lockdown has failed. And the government is facing intense criticism for the humanitarian crisis.
So the government finds it useful to point to “advanced scientific studies” that “show” that the lockdown has saved thousands of lives. Many people tend to get intimidated by differential equations, and so the supposed backing of scientists and formidable mathematics helps the government shut down critics. This is the worst possible use of science and mathematics – to obfuscate the truth, rather than to illuminate it.
These models do not reveal much about the efficacy of the lockdown. But they do reveal a lot about a government that believes in using deception to cover up the consequences of its decisions.
Suvrat Raju is a physicist with the International Centre for Theoretical Sciences (Bengaluru) and a member of the Indian Scientists Response to Covid-19 collective. The views expressed are personal.