The missing national seroprevalence survey has now been found – or so it seems. On July 9, I had discussed the surprising claims that followed completion of the May-June survey to assess the spread of Covid-19 in India. Led by the Indian Council of Medical Research, the survey generated plenty of headlines and speculation, but no preprint or further detail. Now, three months later, a paper in the Indian Journal of Medical Research is available, with 74 authors no less.
With the second national survey now completed, it is unlikely that results of the first will inform many policy decisions. But do we, at least, have a missing piece in India’s Covid-19 story? Do we finally know what was going on with the disease upto May? The short answer is, unfortunately, no. In fact, if the survey holds clear lessons, these are about the conduct of Covid-related research, and not about how India became the world leader in daily Covid-19 cases and deaths. My advice to commentators would be: Do not build any firm narratives around the survey results. There are too many errors, omissions and uncertainties, and any stories built on these foundations could collapse like a house of cards.
Basic problems
Firstly, the scientific integrity of the whole process is in tatters, with the head of the ICMR standing accused of suppressing some of the serosurvey data. The original survey was intended to have two parts: one assessing nationwide Covid-19 prevalence (i.e., how many people had had the disease so far), the other focussed on containment zones in high burden cities. It is the data from high-burden cities which seems to have gone missing, though tantalising snippets were leaked at the time.
We’ll come back to this missing data later – let’s assume the IJMR paper describes the results from the first part of the survey. There is some interesting detail, but critical weaknesses discussed in detail in the supporting material make it nearly impossible to draw firm conclusions.
- There are inconsistencies which confuse the picture. For example, the lower and upper estimates of national Covid-19 prevalence in percentage form do not match the values given as numbers. A technical, but important, inconsistency: an assumed property of one test-kit – its “specificity”, namely the probability that it correctly classifies a negative sample – is inconsistent with the measured data.
- Assumptions and details are left out, making many calculations hard or impossible to check. Some calculations use demographic data which is not given. Some values are used but not given explicitly or referenced: for example, of the delays between infection and the presence of the antibodies, or between infection and death.
- Uncertainties related to the properties of the tests are not fully explored. The estimates of uncertainty explicitly given in the paper seem to underplay question marks over test-kit sensitivity and specificity – the likelihood that the test-kits correctly classify positive and negative samples. Bearing in mind that only 157 positive samples were obtained (out of 28,000), we really need to know how many of these were truly positive; and also how many more could have been missed.
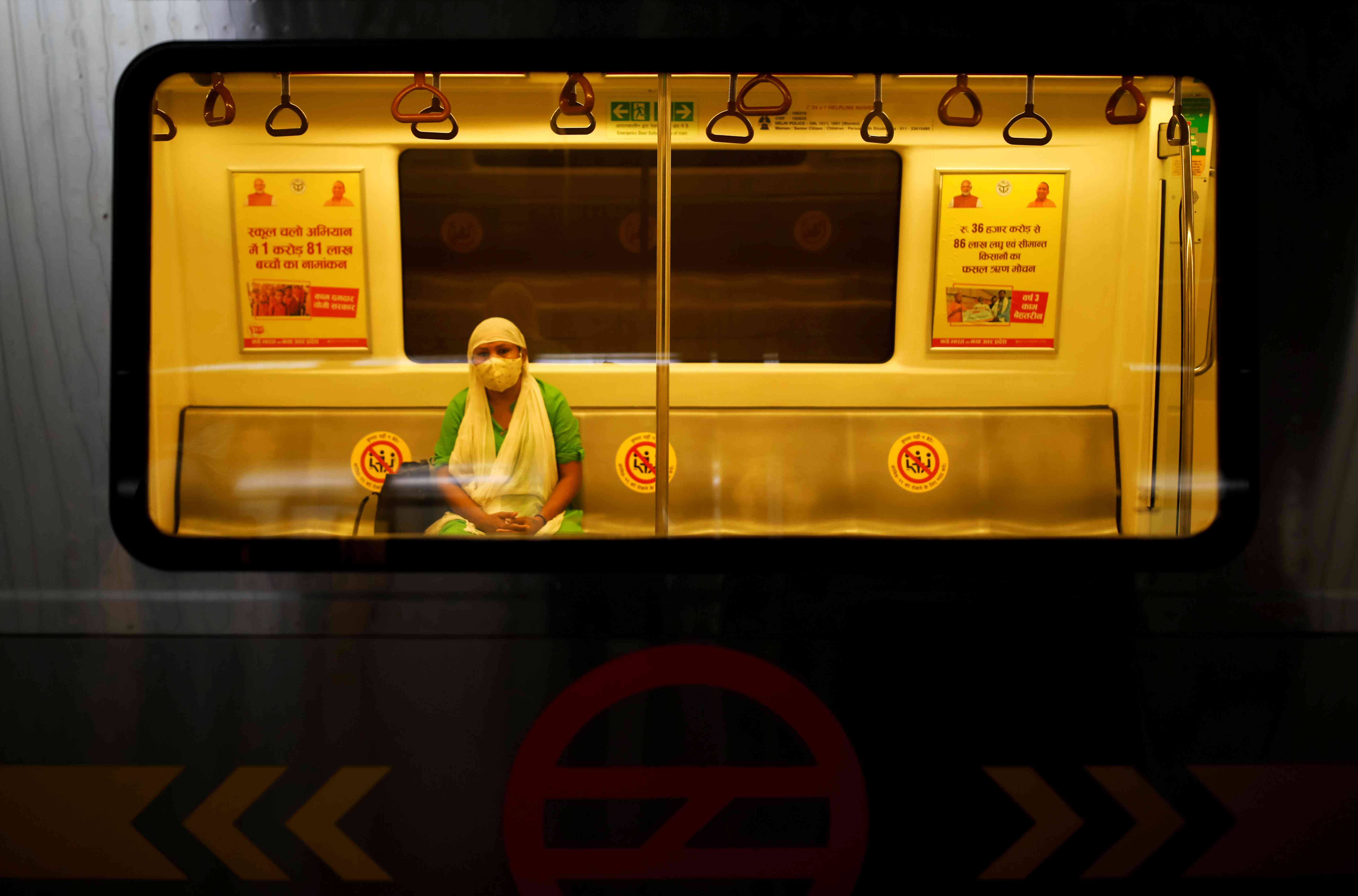
Two competing stories
Almost every claim in the paper can either be taken at face-value or regarded sceptically. Take this: there was apparently no variation in prevalence between “strata” reporting no Covid-19 cases and those reporting relatively high numbers of cases. Does this mean the disease was spreading equally fast everywhere but just not being detected in some places? Maybe, but not necessarily. The test-kit uncertainties are precisely of the kind which confuse such a result.
We are left with two competing stories which read roughly as follows.
1. The face-value story. In April and early May, lockdown had failed to confine disease geographically. Disease was spreading undetected even where there were few or no reported cases – and at the same speed as in regions with high cases. A mere 1 in 125 or so infections had been detected nationally with testing. The same levels of detection today would imply that about half the nation’s population was infected. Either a large number of deaths had been missed, or some unexplained effect was making Covid-19 much less deadly than expected.
2. The sceptic’s story. Prevalence was overestimated on account of incorrect assumptions about the test-kits. Most critical was the untested assumption that the two test-kits used were independent, and hence would be very unlikely to both incorrectly classify a sample. This needed to be explored further. Overestimation of sensitivity and specificity meant that the estimated prevalence was highly unreliable. This largely explains the very low variation in prevalence between strata. The highly variable fatality rates are explained by death undercounting along with overestimation of prevalence, particularly in regions with low and zero cases.
Of course, we do not have to choose between these stories in their entirety. We can accept that case detection and death surveillance were genuinely variable, without believing there was no relationship between infections and detected cases at all. We can also believe that lockdown did slow the export of disease from urban hotspots while acknowledging that some disease “leaked” out.
Fatality undercounting
Perhaps the most important consequence of the survey has been missed in most analyses. And it is not a scientific result in an obvious sense. Rather, for the first time, the ICMR appears to have acknowledged Covid-19 death undercounting – and on a significant scale.
I had speculated from the partial and misleading reporting that followed the survey’s release in June that the calculations seemed to factor in some fatality undercounting. This is now more or less confirmed. The Indian Journal of Medical Research paper suggests that only fatalities for the high case-load stratum where “death reporting was more robust” should be regarded as reliable.
In fact the paper does not even calculate the fatality rates using all the data. But we can do this, and it turns out that the fatality rates the authors consider more reliable are almost three times the raw values that can be calculated from the data in the paper. They seem to be acknowledging that up to two-thirds of fatalities had not been reported by early June.
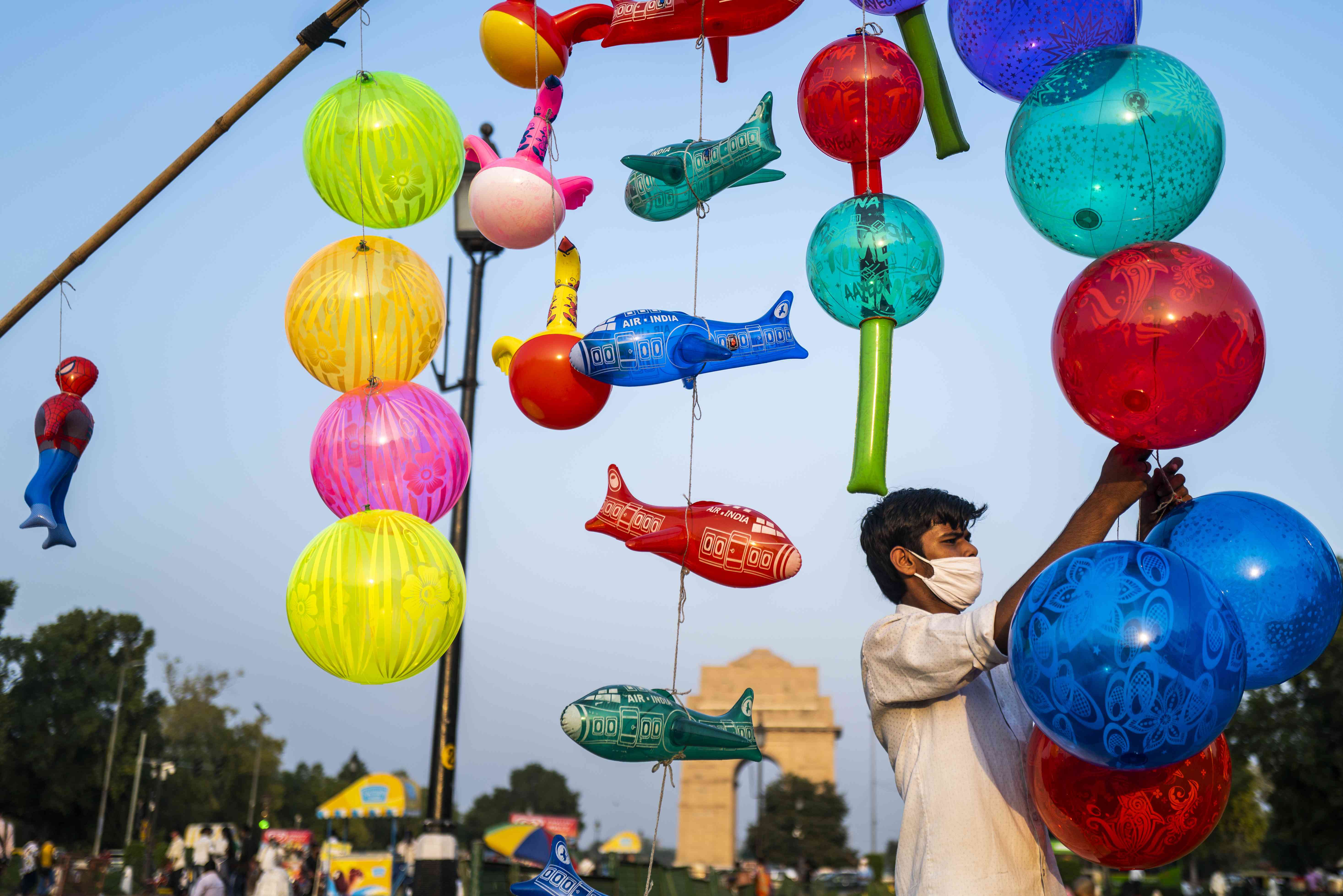
Missing data from high burden cities
Was the hotspot data omitted because it jarred with some narrative? The confused messaging that accompanied the survey completion suggests a desperation for a “success story”. Despite the results, taken at face value, showing millions infected in areas with no reported cases, the ICMR claimed that “lockdown/containment has been successful” and that “India is definitely not in the stage of community transmission”.
People have rightly asked: why suppress the results from hotspots since there is more recent data available for many of these anyway? The hotspot results would be much less vulnerable to test-kit uncertainties, and combined with later survey results might give valuable insights into the disease over time. Whatever the back-story, it seems clear that suppressing some data is about managing headlines even at the cost of the integrity of the whole exercise, and the ICMR as a whole.
Conclusions
Ultimately, the survey set out to do something fraught with difficulty – measure a small prevalence using available kits with uncertain properties. Previous studies had already foreshadowed the difficulties – the IJMR paper even references one such study. The survey and analysis made some attempt to deal with the difficulties, but these were not adequate, and we are left with only the shadows of results. Instead of exploring the key uncertainties further, and discussing them transparently and fully, the report understated them.
Ultimately, we still do not know if lockdown confined disease geographically, or how much disease reached rural India in the early days. We don’t know for sure if national disease detection was much worse than in hard-hit cities like Delhi and Mumbai. But, at least, we do have a guarded acknowledgement that Covid-19 death reporting may not be uniformly robust.
Murad Banaji is a mathematician with an interest in disease modelling.