Punjab’s surging Covid-19 cases and the high mortality rate has prompted the Union health ministry to deploy central teams to assist the state and the Union Territory of Chandigarh in containing the infection. Punjab had 19,096 active cases of Covid-19 as of September 12, 53,308 have been recovered so far and 2,212 have died. A sudden spike in August has led to a more than 250% increase in cases and a 345% increase in deaths (1,332) since the end of July.
Findings from research by Delhi-based think tank Centre for Policy Research could hold some clues to what could have happened in the state, which has a population of 2.8 core.
Centre for Policy Research is working alongside the government of Punjab to study the asymptomatic transmission of Covid-19 in the state. They recently put out a research note with their early findings.
Eighty-one per cent of Covid-19 transmissions in Punjab came from asymptomatic people and symptomatic people contributed to only about 15% of infections, according to contact-tracing data in Punjab, analysed by the Centre for Policy Research.
The fact that asymptomatic transmission is so dominant in the state indicates that the testing strategy needs to change–from contact-tracing only of infected patients to actively finding new cases through a wider, population-based surveillance – the note said.
Although Indian authorities have not declared community transmission in many states, “it is increasingly likely that this is the case”, the researchers noted, raising yet another compelling reason for testing criteria to stop focussing only on those who present with symptoms.
In an interview with IndiaSpend, Jishnu Das, who is part of the Covid-19 research group at Centre for Policy Research, talks about their research in Punjab and on the larger issue of asymptomatic transmission.
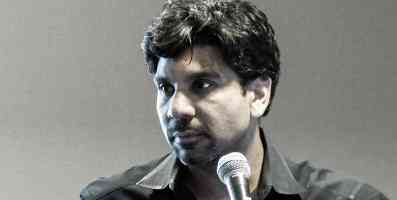
Excerpts from the interview:
Your research shows that testing strategies in Punjab need to shift from contact-tracing of infected cases to active case-finding through population surveillance, given the dominance of infections from asymptomatic patients. Active case-finding, however, is based on screening for the symptoms of Covid-19, but when there are many asymptomatic carriers, the model is incomplete. This brings us to a three-pronged question:
(i) When is a good time to start screening the population and community to catch asymptomatic cases – when there is “dominance of infections from asymptomatic patients”?
(ii) This would mean that a state should primarily have an active surveillance programme but, as a subsidiary project, run passive surveillance to see if it is reaching that tipping point–of high infections from asymptomatic cases–so as to switch from active testing to community testing. Is that correct?
(iii) How should a state – and a country of India’s size – with limited resources figure out that sweet-spot?
These are all the right questions and the basic fact is that we have principles on which these can be answered, but no specifics. The reason why we do not have specifics is because, in general, we have not followed a path of asking the right questions and then stepping back and saying: “Ok, how do we answer these”. So, we are working with data that are not perfect with considerable uncertainty on a number of fronts – and certainly not designed to answer these questions.
If we had known from the beginning that many cases will be asymptomatic and asymptomatic people will transmit the illness, then we could recalibrate the potential health outcomes from Covid-19 (after all, if asymptomatic people never fall seriously sick, they will not need healthcare and certainly not hospitalisation) and emerge through an iterative learning agenda to see how asymptomatic people could be detected early.
So, the right answer to your question depends, in part, on whether there is a well-calibrated strategy that will allow us to detect asymptomatic infections in the first place. If there is not, then even when asymptomatic infections are dominant, community testing may not be cost-effective. Our research note highlights the conditions under which even simple random sampling in the community may be a good idea.
India has changed its testing protocol many times, gradually increasing the number of people who can be tested. Is it necessary to have dynamic testing criteria? Is it necessary to have all-India criteria or state-wise? Why should it differ from state to state?
At the moment, Punjab is doing four types of things: therapeutic testing for Covid-19, serological surveys to detect the fraction of population that have had Covid-19 in the past, syndromic surveys of ILI [influenza like illness] and SARI [severe acute respiratory infections] patients, for instance, and testing in special populations, where the definition of special populations has changed over time, as you have noted. So, in some sense, there is already the idea of community testing.
The key question is whether the predictors of infection that they are assuming – influenza like illness or severe acute respiratory infections predominantly and special populations in some cases – are the most cost-effective ones.
What our research points out is that these are not very good predictors of infection and there is really no active effort to find better risk predictors.
Your question about when is the sweet-spot to switch from passive to active surveillance is exactly the right one. We need to figure out how much community testing to do and whom to test. The two questions are related because if we can figure out whom to test in an appropriate manner, this directly increases the efficacy of community testing.
So, yes, it is absolutely critical to have dynamic testing criteria, but that testing criteria has to be based on what we are learning about the disease so that the “dynamic” part of it is driven by what we have learnt.
For instance, we did not know earlier that loss of smell and taste are two potential symptoms. So, we should “dynamically” change our screening criteria to include these. Similarly, we have yet to “learn” the behavioural markers of infection – are certain occupations or groups of people (perhaps those who meet lots of other people) more likely to be positive early in the epidemic? When prevalence is low, what group should act as the sentinels of an incoming epidemic? All of these questions need to be learnt. The interesting part is that this is not difficult to learn and it does not take a lot of time, but it does require a structured approach to testing and learning, which we have not taken thus far.
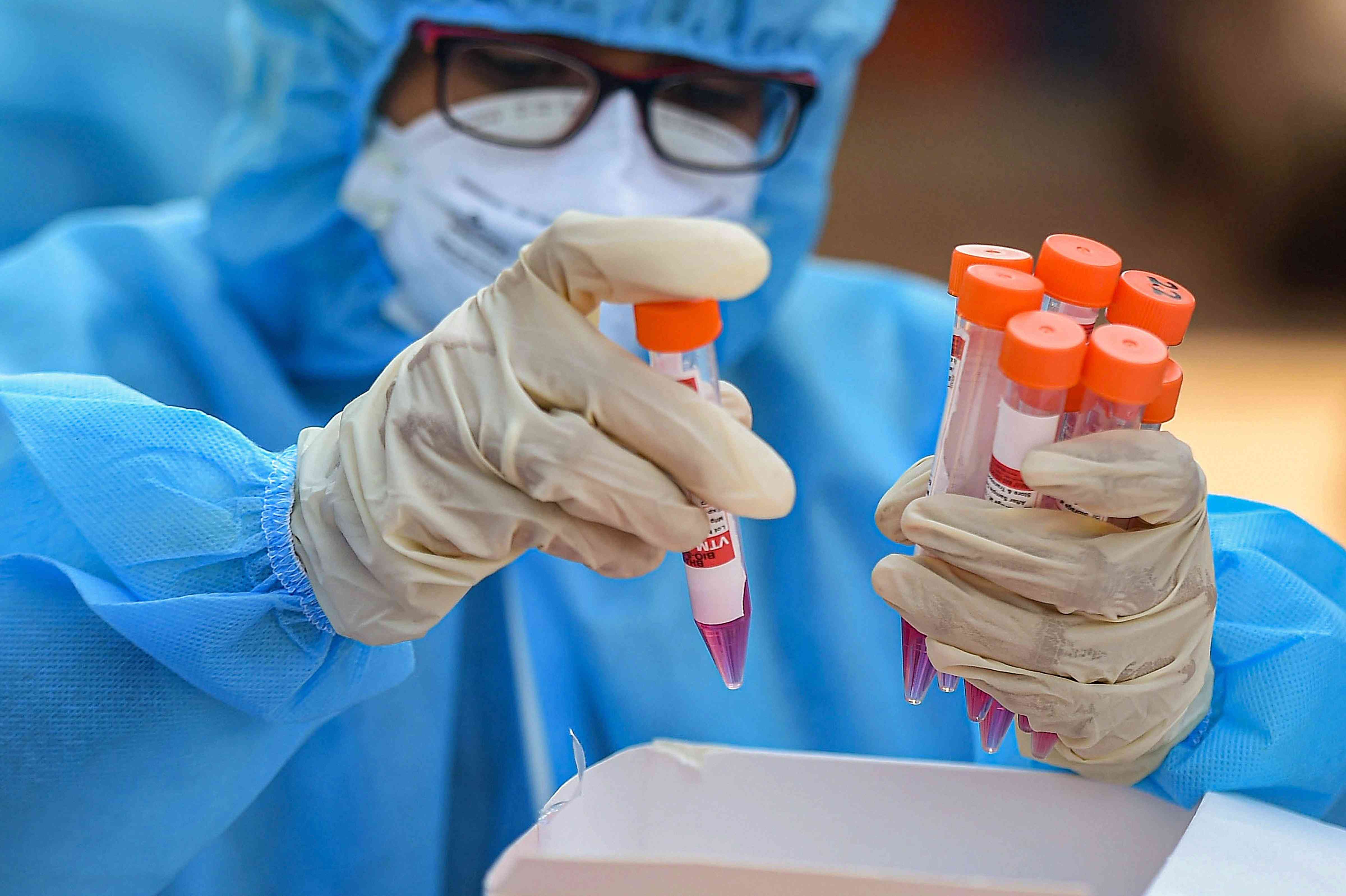
What is the cost-effectiveness of testing widely to catch asymptomatic cases? What are the qualitative or quantitative arguments in favour of or against testing widely for asymptomatic cases?
On cost-effectiveness, all of it will come down to precisely how well we are able to screen people for testing, especially those who are asymptomatic. Our simulations compare one particular model – random sampling in the community – and we show that even at fairly low overall prevalence, this may be a better way to test compared to symptomatic screens. But this does not answer your deeper question of whether you should do community testing at all.
So, my take is very much that this is something we should spend some money and time on learning quickly and through different approaches to the samples selected. Again, it is not a lot of time and money if done smartly.
Given that tests are not accurate and also that a person testing negative today could actually pick up the virus tomorrow, why should one test asymptomatic cases? Should an asymptomatic person be tested over and over again? Your research, for example, suggests testing those who play an active role in the community – such as shopkeepers – who could catch or spread the infection most. Does your model suggest that they should be tested routinely?
This general question of how often people should be tested depends on what we want to use the tests for. For instance, in an area with low prevalence, if we can “learn” who the early infectees are (perhaps health workers or police?), then a sentinel surveillance system among them may work well. Or, we can look at areas where there has been a lot of population movement and mark these as places where there should be more testing. So, your intuition is correct – people who have a high chance of catching the infection early should be tested more often. But we need data to know who the people who catch the infection early are.
Now that the infection is overwhelmingly widespread in India, is it even possible to do surveillance, to break the chains of transmission when it does seem that the virus has overtaken human efforts of stemming it?
Sure. There are ways of doing things more effectively, even at high prevalence levels. We have a paper on that coming out soon, so you will have to wait a bit. But again, a lot of this comes down to whether we have an active learning agenda in place to get at this. Remember that our positivity rate at the country level is still at 8%, so for each positive person we are detecting, we are testing 12 people. Even increasing the efficiency of that by a bit would be huge.
How has your study taken care to not mix up “asymptomatic” and “presymptomatic”, given that it is possible that someone asymptomatic today turns symptomatic tomorrow?
No, we have no way of knowing from these data who is asymptomatic versus presymptomatic, since that would require the further merging of these data with hospital records for each person. We do not have access to these. Having said that, [there are] two things. There was one study in India that showed that a lot of asymptomatic patients at the time of testing continue to remain asymptomatic throughout their illness. Second, since these are people who are positive at the time of testing, for infection dynamics, it may matter a bit less – what is critical is to catch them at the time they are infectious.
This article first appeared on IndiaSpend, a data-driven and public-interest journalism non-profit.