Since the beginning of the Covid-19 pandemic, there have a been a large number of seroprevalence surveys – or “serosurveys” for short – to estimate how many people have been infected with the disease. These involve sampling populations at national, state, district, city, or even ward level, to estimate “seroprevalence”, that is, to find how many have antibodies to SARS-CoV-2, the virus responsible for Covid-19. With a third national serosurvey now completed, what are some of the lessons from past serosurveys?
Serosurveys have provided insights into the pandemic that aren’t readily visible in data on cases or recorded deaths. Remember that “cases” are recorded infections, confirmed using some test such as RT-PCR, and we expect these to be the tip of the iceberg of total infections; serosurveys on the other hand should indicate the true fraction of the population who have been infected.
The surveys have taught us that urban spread – particularly in city slums – can be very rapid indeed, and that new surges are possible even when a large part of a population has had the disease (as in Mumbai and Delhi). They have highlighted highly variable disease surveillance across the country, with weaker surveillance in some states, in rural areas, and in city slums. And they have indicated that fatality recording is probably extremely variable.
But the surveys also leave many questions unanswered. Sometimes they tell us less about the disease and more about the interaction between science, media and politics. Misleading narratives often accompany the results, and important messages can get obscured by political considerations and poor reporting.
Uneven spread: urban poverty and marginalisation
Several of the surveys have highlighted two related points: that Covid-19 spread and Covid-19 detection can both be very variable.
Mumbai’s and Pune’s surveys demonstrated that poor housing is strongly associated with the rapid advance of Covid-19 in cities. Delhi’s third serosurvey found that disease had spread more slowly in planned colonies. The higher prevalence in urban slums was also noted in both second and third national serosurveys. In fact, all the available data suggests that housing poverty is a key predictor of how fast COVID spreads in cities.
But some city surveys have failed to report results broken down by dwelling. One immediate lesson is that any Covid-19 survey which fails to factor in housing, risks incorrectly estimating the extent of disease.
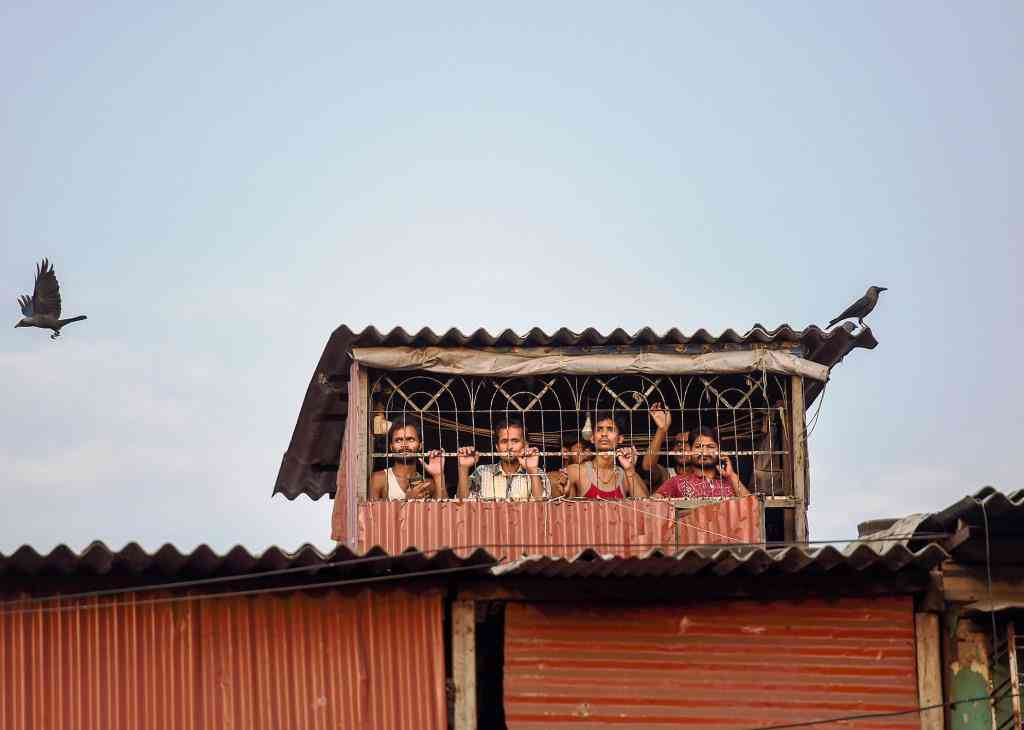
Rapid spread in slums points at some deeper questions. Poor housing and shared facilities are likely to directly accelerate disease transmission. But housing is also associated with occupation, access to healthcare, and poverty quite generally. All of these are in turn associated with caste and community – this is important because marginalisation, stigmatisation and discrimination could well accelerate the spread of Covid-19 over and above poverty, as we tend to see in international data.
The question of how multiple related factors influence Covid-19 spread has largely been ignored in most analysis of the Indian epidemic: crowded housing, high risk occupations, marginalisation, and the lack of an effective social security system forcing people into high-risk situations. Ideally serosurveys should be gathering data granular enough to disentangle the effects of different layers of poverty and discrimination on the spread of the disease.
Uneven spread: a rural-urban divide?
Rural spread has, in general, been slower than urban spread. This was observed in the second national serosurvey, in the third national serosurvey, and also in several local serosurveys. A survey in Haryana in October found 11.4% with antibodies in rural areas as against 19.8% in urban areas, with major cities reporting seroprevalence of upto 40%. The trend for higher seroprevalence in more urban districts was also seen in districts surveyed in Chhattisgarh.
But these trends are not uniform. Amongst the districts in Bihar covered by the second national survey there was a trend for more rural districts to see higher seroprevalence. The heavily rural district of Madhubani saw seroprevalence of 23% by late August.
Thus, although urban areas tend to see more infections, we should not rush to assume that rural spread is always slow. Rather than focussing on a broad rural-urban divide, it seems more important to understand how patterns of rural development, including connectivity, and the nature of housing, employment and travel, might contribute to accelerating rural spread.
Uneven detection: how many infections get picked up in testing?
If there is one overwhelming message from the surveys it is that the detection of infections is much higher in some settings than others. The percentage of infections that get recorded as cases varies by orders of magnitude between states with relatively good detection like Delhi and Chhatisgarh, and poor detection like Bihar.
Even within cities there are huge divides. In Mumbai, detection of Covid-19 infections in non-slum areas was an estimated 8 times higher than in the slums. The slums saw a sharp rise and fall in infections over April and May, almost totally obscured in the city’s case data. Something similar appears to have happened in Pune where hard-hit slum wards had in fact generated relatively few cases.
Poor detection in the slums may partly reflect younger slum populations and consequently fewer severe cases. But there are indications that it is about more than this, and that testing has been generally much poorer in the slums.
Is there a rural-urban divide in detection similar to the slum/non-slum divide? In both Bihar and Chhattisgarh the serosurveys indicated better detection in more urban districts, although this trend was more pronounced in Bihar. In Haryana, on the other hand, a quick check finds no clear relationship between the level of urbanisation of a district and the fraction of infections detected in testing.
These results suggest that what we see as a rural-urban divide in detection might simply reflect levels of rural/urban development and poverty.
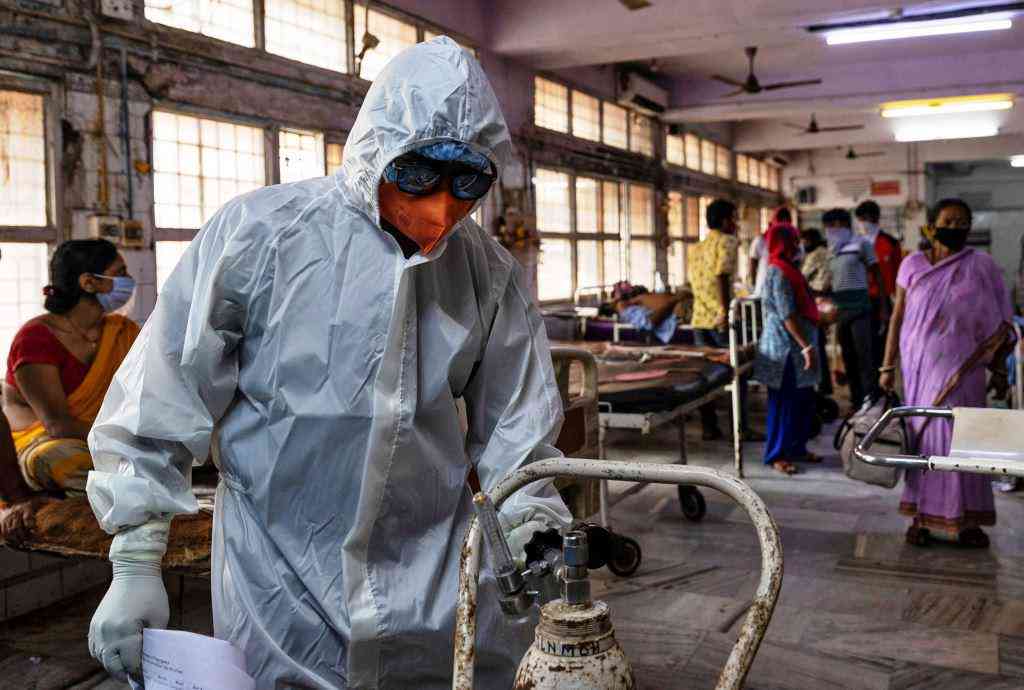
Death reporting may be extremely variable
Although the serosurveys don’t tell us directly about deaths, they do teach us that we should be quite sceptical about reported Covid-19 fatality figures.
It is interesting that despite widespread reports of Covid-19 fatality undercounting nationwide, the first “official” acknowledgement of its occurrence came from an analysis of serosurvey results. The paper reporting on the first national serosurvey, many of whose authors were associated with the Indian Council of Medical Research, acknowledged that death reporting was not robust in many areas. As a consequence, the authors chose to discount a large part of the data when estimating fatality rates.
A striking example of problems with fatality data is seen in a comparison of results from Bihar and Chhattisgarh. Narratives about Bihar’s “success” in controlling the spread of Covid-19 were founded on relatively low numbers of recorded cases and deaths. But seroprevalence data from the second national serosurvey showed that the state was recording only a tiny fraction of its infections. The almost total absence of Covid-19 deaths from regions of the state with high levels of infection raised the question of how many deaths may have simply gone unrecorded.
Meanwhile, serosurveyed districts in Chhattisgarh reported lower seroprevalence values but many more fatalities. At face value the chances of someone with COVID-19 in Chhattisgarh dying seemed 15 to 30 times higher than in Bihar. The most plausible explanation was that Chhattisgarh was recording a much higher proportion of its fatalities than Bihar. Yet, in the narrative accompany the latest Economic Survey, Bihar figures as a success for its lower than expected cases and deaths, whereas Chhattisgarh is shamed for what amounts to better surveillance.
But... can we trust serosurvey results?
We’ve already made a number of claims based on serosurveys, but there remains the prickly question of how much we should trust them. Could they be over- or under-estimating the spread of disease?
We should, indeed, treat the results critically. Very uneven spread complicates the task of sampling a population to determine how many have been infected. Timing, the choice of antibody test, and sampling methodology can have huge effects on the results.
Consider two different serosurveys in Karnataka which threw up highly divergent results. The first, between June and August, found 47% of those sampled had developed antibodies to SARS-CoV-2, while the second, during September, found only 16% with these antibodies. Whether the explanation lies in different approaches to sampling, testing methodologies, or elsewhere, this example illustrates that seroprevalence values cannot always be taken at face value.
There are several particular reasons to be cautious about serosurvey results, discussed next.
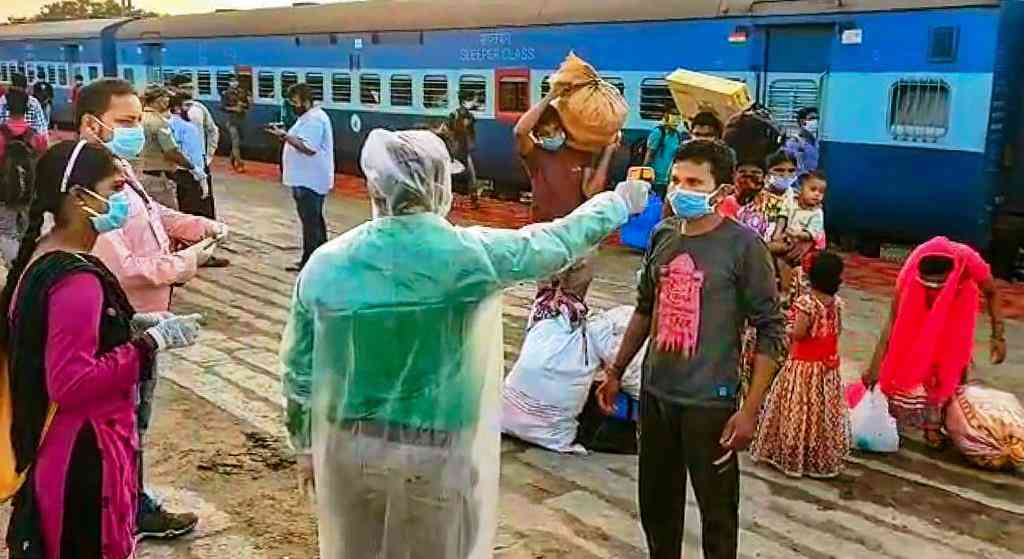
Serosurveys may miss some past infections
A fraction of people who have had Covid-19 – especially those infected several months prior to the survey – may not have measurable antibodies to the virus. Antibody responses vary between individuals, and antibody levels naturally diminish over time after recovery from an infection; but whether they become undetectable seems to depend strongly on the test-kit used. Unfortunately reports often do not even mention which test was used.
Delhi’s first four serosurveys reported, approximately, 23%, 29%, 25% and 25% seroprevalence between June and October – despite many Covid-19 cases and deaths during this period. The results strongly suggest decreasing detection of old infections over time. This was confirmed in the fourth serosurvey: a sample of recovered Covid-19 patients were tested and antibodies could not be measured in about 44% of this group.
A fifth Delhi serosurvey, apparently using a different antibody test with greater sensitivity, reported much higher seroprevalence of 56%.
Similarly, Mumbai’s second serosurvey found a decreased seroprevalence amongst slum-dwellers compared to the first. The extent of the drop made it highly unlikely to be a consequence of sampling errors. Closer examination found that the test used was particularly vulnerable to missing old infections.
Transparency, delays and spin
The goal of serosurveying during an epidemic is surely to help manage the epidemic – and yet this goal has often been undermined by a failure to share much of the data and an obsession with putting a positive spin on the results.
The first national serosurvey could have provided some valuable insights during the early days of the epidemic; instead the whole event was a fiasco. There was a press conference in June with some headline figures but no useful or credible detail, followed by silence until a flawed paper appeared in September.
By this time, Covid-19 had well and truly moved on. Moreover, some of the most potentially valuable data from containment zones was never released by the ICMR. Ultimately, with the long delays, methodological flaws, and extremely poor transparency, this survey now appears as a huge waste of time and resources.
Delhi has surveyed prolifically – there have been five serosurveys in the city so far. But the process has again been marred by poor planning and transparency. Different antibody tests and different approaches to sampling were used across the surveys. Very little technical documentation was made available when the results were reported, although in December 2020 a preprint with more detail on the second, third and fourth surveys appeared.
It is likely that transparency improves when academic bodies are partners in the survey, as in Mumbai and Pune, although even in this situation government tends to call the shots.
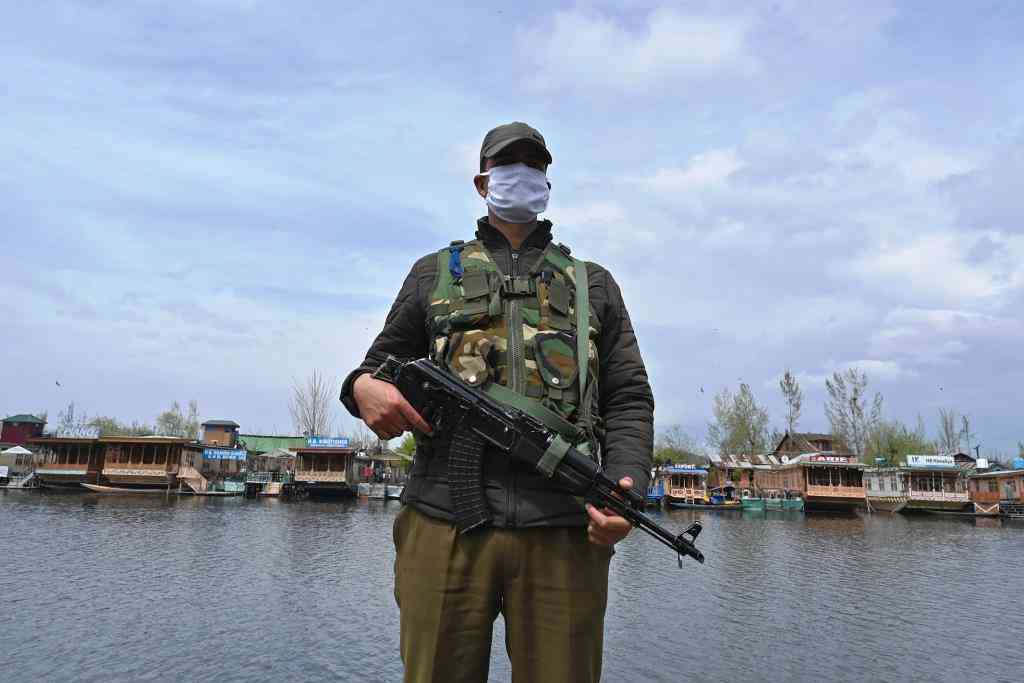
Reporting on serosurveys can be very poor
Reporting on serosurveys can be of poor quality. There can be glaring inaccuracies, the data is rarely put in context, and the right questions often remain unasked.
The fact that governments and “sources” drip feed information – sometimes inaccurate information – to the media is part of the problem. After the first Delhi serosurvey, “sources” originally claimed that the positivity rate was around 10%, although this figure later turned out to be 23%. Early reports on Delhi’s third serosurvey claimed that 33% of those tested had developed antibodies, although later this turned out to be 25%.
On top of this, media houses sometimes generate wild claims of their own. An extreme example is a report from Zee News which claimed that 69.4% of people in rural India had been infected with Covid-19 by the time of the first national serosurvey. The same claim was repeated by other media houses. Actually, fewer than 1% of samples tested were found to be positive, but the confusion arose because 69.4% of the positive samples had come from rural areas.
Aside from inaccuracies, much reporting on serosurveys simply repeats claims by authorities without any critical appraisal. After the first national serosurvey, the ICMR simultaneously indicated that there had been very many undetected infections by the end of April, and insisted that there had been no “community transmission” by that point. Both claims were reported widely but few reports noted the contradiction. Various others dubious claims were reported uncritically, including an estimate of fatality rate which did not appear to tally with other data, and never appeared in the journal paper eventually reporting the results.
Sometimes critical information is missing in reports. Data shared by the private firm Thyrocare in July indicated that 15% of those who underwent antibody tests nationwide during a 20-day period had developed antibodies to SARS-CoV-2. Many reports on the Thyrocare survey failed to mention that it was not a randomised survey: participants had chosen to get tested or were obliged to do so by work or residential societies, likely inflating the positivity rate.
Some outlets even reported the data without mentioning its origins at all. Indeed, the results of this survey were at odds with the second national serosurvey carried out during August and September, which reported only 6.6% seroprevalence nationally.
It is quite common for high seroprevalence values to be accompanied by positive – and generally uninformed – spin about herd immunity, or low fatality rates. Such “good news” stories ignore widespread underreporting of fatalities, the lack of reliable data on the toll of the pandemic in excess mortality and long term health consequences, and the dangers of accelerated antigenic drift, leading to the development of new virus strains, in populations with high prevalence.
Broad conclusions
Although the data is patchy, seroprevalence data from around the country has deepened our understanding of India’s Covid-19 epidemic. Ignoring this data and focussing exclusively on recorded cases and deaths, as done for the Economic Survey 2020-’21, can give rise to entirely incorrect conclusions, rewarding poor disease surveillance.
Some clear and consistent patterns have emerged from the surveys, such as more rapid spread in urban slums, and weaker detection of disease where poverty is high, and access to healthcare is likely to be poorest. We find big differences between states, and a rural-urban divide in spread and in detection of infections, whose reasons need further study.
However, the serosurveys have raised questions as much as answered them. Huge variations in apparent fatality rates hint that Covid-19 fatality reporting is weak to nonexistent in some parts of the country. A large number of deaths may have gone “missing”, especially from states with poor registration and medical certification of deaths. If a narrative of low fatality was not politically convenient, questions around mortality would surely have spurred further investigation.
The wide variation in measured seroprevalence values in different settings hints at how the disease interacts with patterns of travel, work, housing, and marginalisation. Unfortunately the data needed to follow up on these questions is often missing. Age- and gender-based information are routinely gathered in serosurveys, but data about income, occupation and living environment are often not. And no survey has, to my knowledge, examined how caste and community might be associated with seroprevalence.
Of course there are valid fears that such data could be used to further stigmatise communities, but this should not be used as an excuse to avoid addressing basic questions around how Covid-19 has interacted with existing inequality and discrimination.
While seroprevalence data is in general a more reliable indicator of disease spread than case data, it is not free of problems. Analysis needs to factor in the fact that antibody responses take some time to develop after infection, and that some tests become less sensitive to old infections over time. Ideally, we should always check if serosurvey conclusions are consistent with other epidemic data, and whether results could be affected by how a population was sampled or the timing of the survey.
Finally, it is important to bear in mind that what we get to see after a survey is what government (and, occasionally, private bodies) choose to share, and that the information may be incomplete, inaccurate or misreported. Only a few surveys have been accompanied by technical documentation when results are released to the media. Political considerations, incompetence, and a fear of independent scrutiny seem to underlie many decisions on what data is released, and when.
Murad Banaji is a mathematician with an interest in disease modelling.