On April 15, Indian government released a list of 170 districts across the country designated as coronavirus hotspots. Of India’s 735 districts, 49% did not have any infections. The remaining 377 districts were categorised as follows: 170 of these districts are hotspot districts (red and orange districts in Figure 1), and the remaining 207 are non-hotspot districts with some infections.
The 170 hotspot districts were further categorised into hotspots with cluster outbreaks (less than 15 cases) and districts with large outbreaks (more than 15 cases or multiple clusters). From April 20, districts with no infection have been allowed to resume some economic activity. The rest of the country will likely see a staggered resumption of economic activity with the lockdown being the strictest in the hotspot districts.
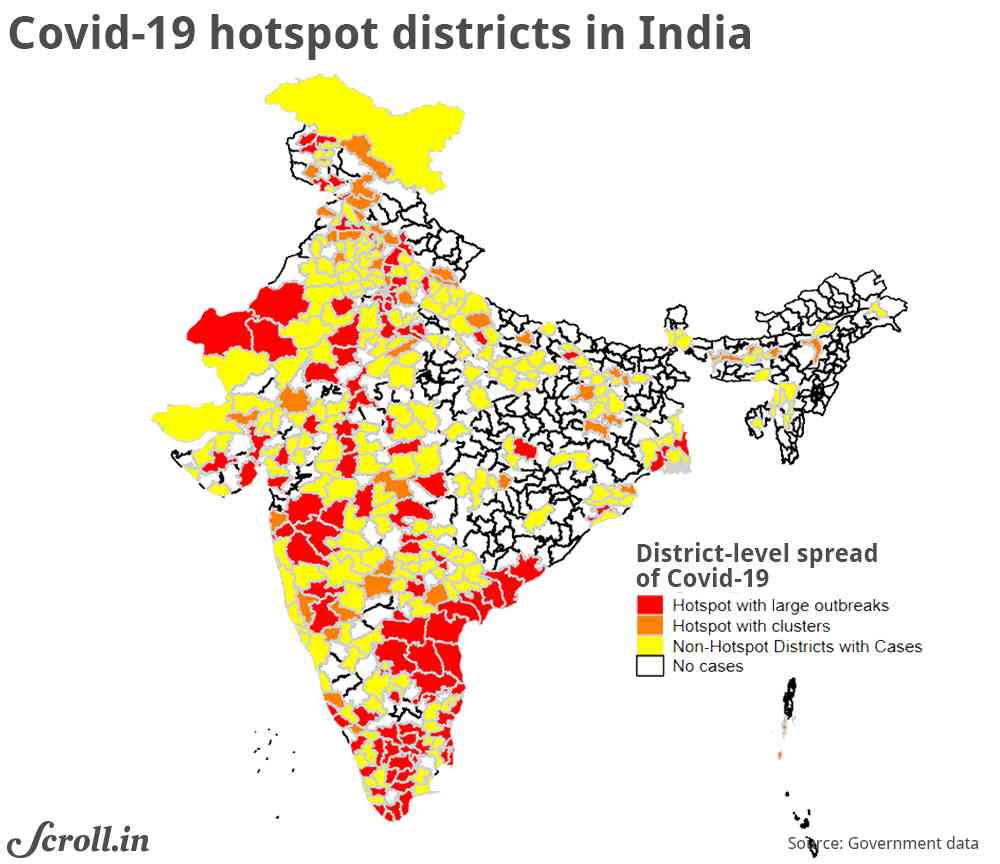
What do districts in these various categories look like? Which category is poorer? Which category carries a larger burden of comorbidities – additional health conditions such as chronic kidney disease, diabetes or hypertension that put residents in the high-risk category for Covid-19? Do residents living in hotspot districts have easier access to handwashing facilities?
In this article, we describe the four categories of districts along two broad dimensions: socio-economic characteristics and burden of Covid-related morbidities. The objective of this exercise is not to predict infection or death rates, or the possible evolution of the disease. Instead, our exercise describes hotspot districts and is a first step towards identifying those that have a larger concentration of at-risk populations. Understanding the characteristics of non-hotspot districts can also be vital to prepare for a potential outbreak and potential hurdles in providing social protection.
We use geocoded data from various sources, including Socioeconomic Data and Applications Center and the fourth round of the National Family Health Survey. We have combined these data with the latest district boundaries for the country. Since the National Family Health Survey data was collected in 2015-’16, for variables not drawn from this survey, we use data from 2016.
Population characteristics
Hotspot districts with large outbreaks are mainly urban. These hotspots have a high population density (an estimated average of 2,589 people per square km in 2016).
By contrast, districts with no infections are largely rural. In districts with no infections, the average share of the urban population is 19%. In districts with some infection, the proportion of people who are older than 65 is the same as those in districts with large outbreaks (Figure 2).
Therefore, the current burden of Covid-19 in India is higher for denser, more urban areas. These areas also have a larger share of older people, who are more at risk for critical illness.
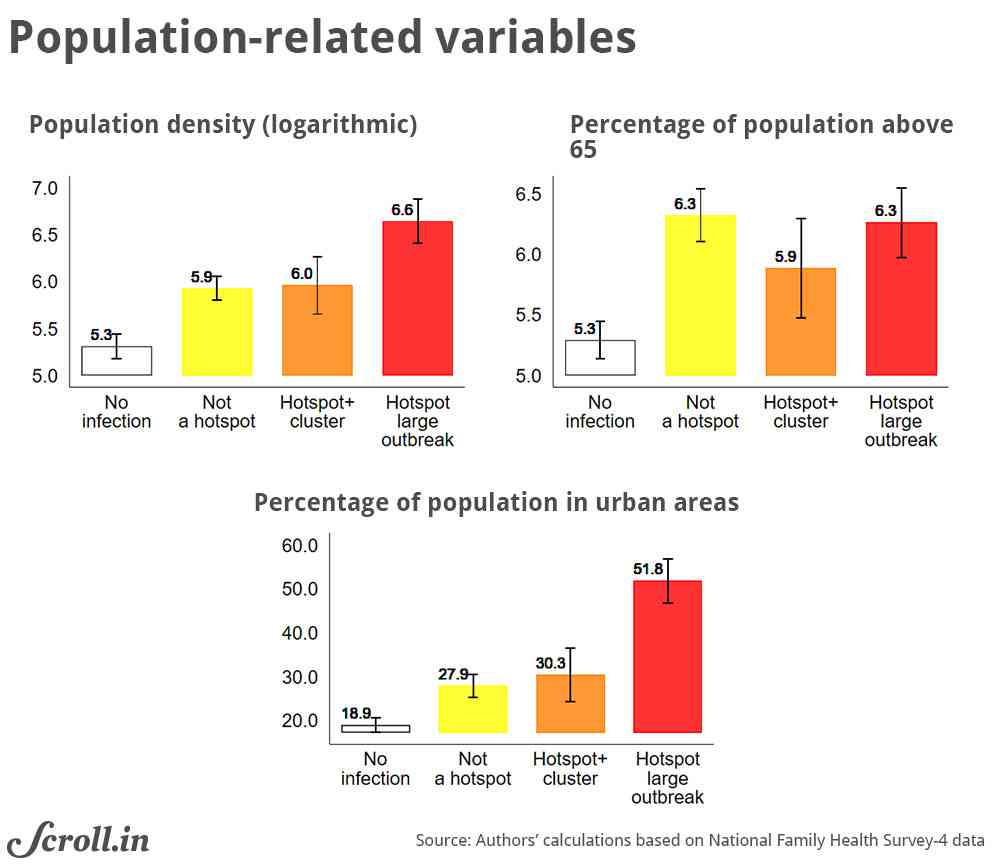
Economic characteristics
Over 30% of the population in hotspots with large outbreaks are among India’s one-fifth most wealthy people and only 7.2% are from the country’s one-fifth poorest people. As things stand now, the wealthier parts of the country are worse hit (Figure 3). This pattern suggests that residents in the current hotspot districts may have a higher financial buffer to deal with the consequences of the lockdown.
If infections spread rapidly to those districts that are currently not severely affected, the consequences for both illness and livelihood management will be more challenging.
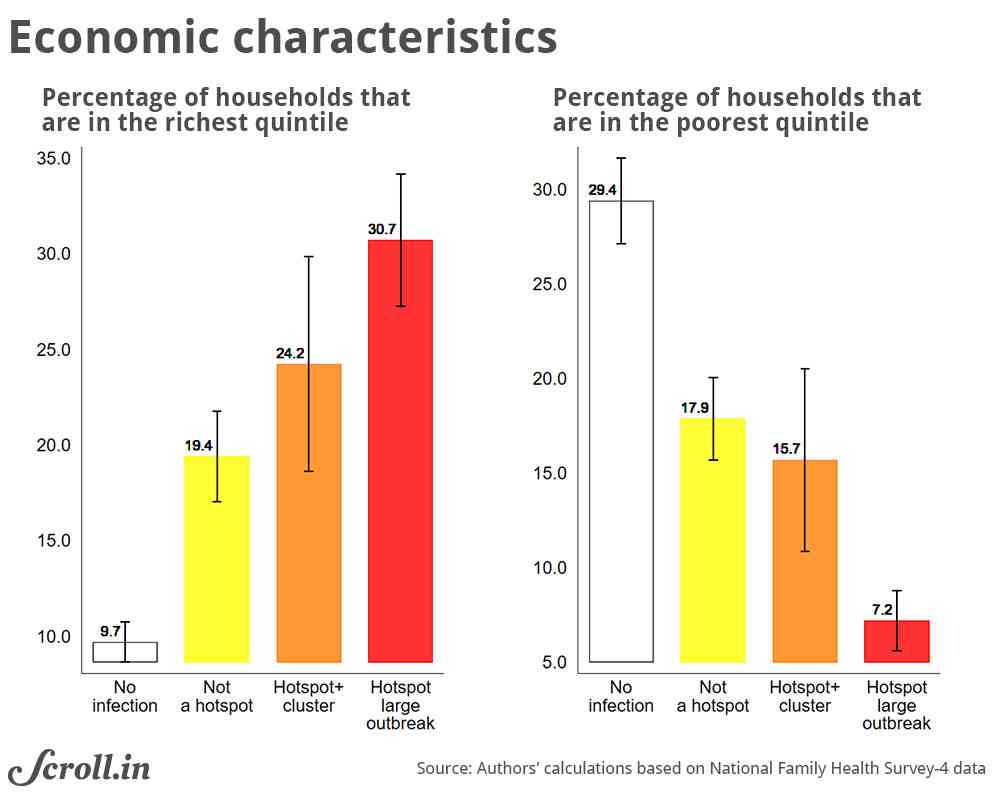
Potential cccess to Aadhar-linked transfers
On April 1, the government said that any financial assistance would be disbursed through Direct Benefit Transfer systems, which will be managed through the architecture that links beneficiaries’Jan Dhan accounts, mobile phone numbers, and Aadhaar numbers. Beneficiary accounts have to be linked with Aadhaar – the government’s project to give every Indian resident a 12-digit biometric-based unique identity number – to receive government transfers under this system.
Using the individual-level Aadhaar card ownership from the National Family Health Survey, we can see that the districts with positive infection rates had a significantly higher Aadhaar coverage in 2016 (Figure 4).
Fourteen per cent of those who did not have an Aadhar card in hotspot districts were also the poorest 20% of all Indians. While the coverage of Aadhaar has increased tremendously since 2016, the neediest populations in hotspot districts are likely to bear the burden of being excluded. Relatively lower coverage in currently unaffected districts is still a concern in case infection spreads.
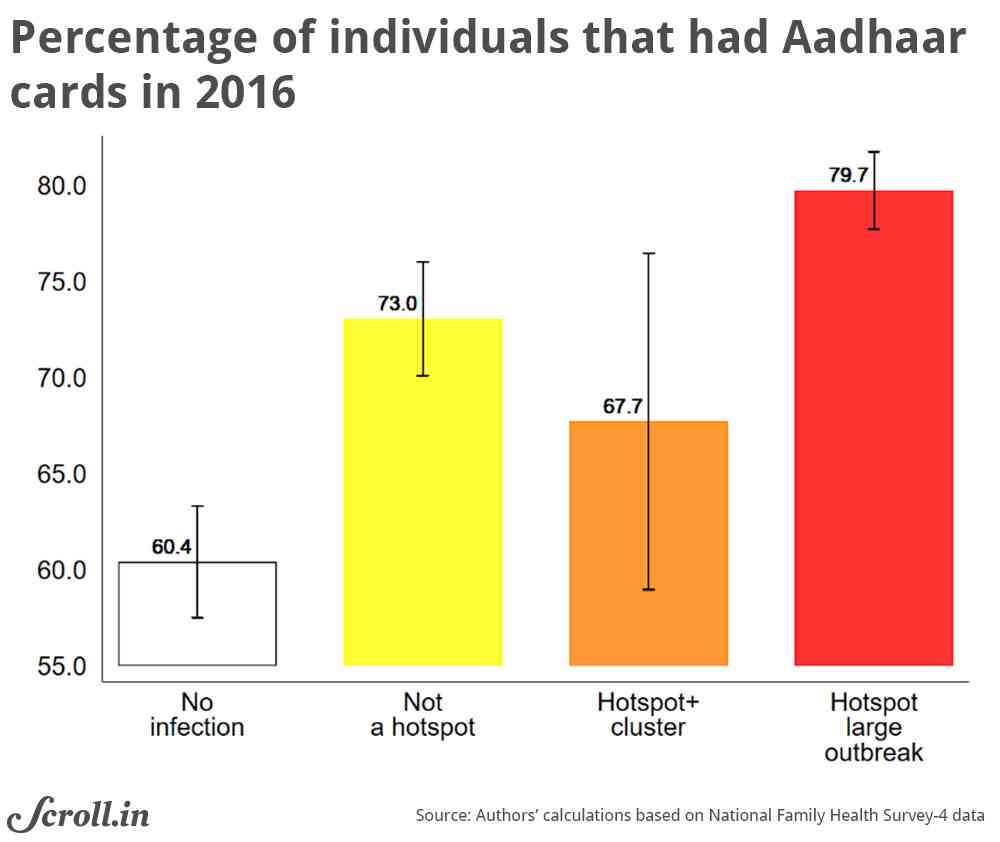
Access to handwashing facilities
Frequent hand washing is considered the most effective and one of the easiest ways of containing the spread of Covid-19. Of course, one needs to have access to water and soap to wash their hands. With strict social distancing norms, one should be able to access water without leaving the homes.
However, access to water at home in India was consistently low in all districts. In 2016, while 88% of households reported that they practice handwashing, only 14% households in hotspot districts had access to piped water or a water source that was within the household premises.
Moreover, the burden of fetching water was disproportionately borne by women and young girls, putting women at a higher risk of exposure to the infection (Figure 5). If the infection spreads, it will be even more difficult to follow handwashing norm and practice strict social distancing, especially for women.
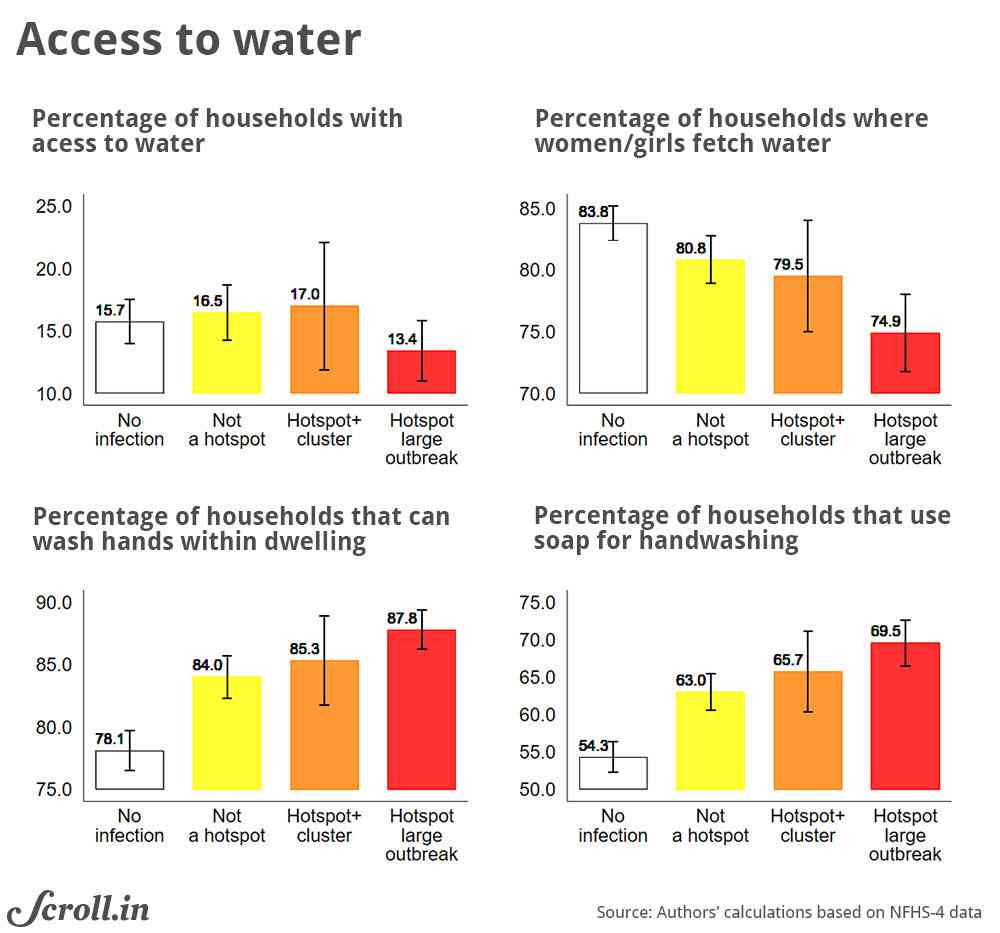
Covid-related comorbidities
We know from early research that certain preexisting conditions, such as diabetes and hypertension, are a risk factor for critical patients. We use data from women’s interviews in the fourth round of the National Family Health Survey to look at the distribution of diabetes, hypertension, and obesity across districts.
Covid-affected districts bear a larger burden of these comorbidities, with the burden associated with obesity being the largest. Since obesity is associated with a higher vulnerability to viral infections, perhaps including Covid-19, its incidence may be a useful predictor for identifying hotspot districts with larger at-risk populations.
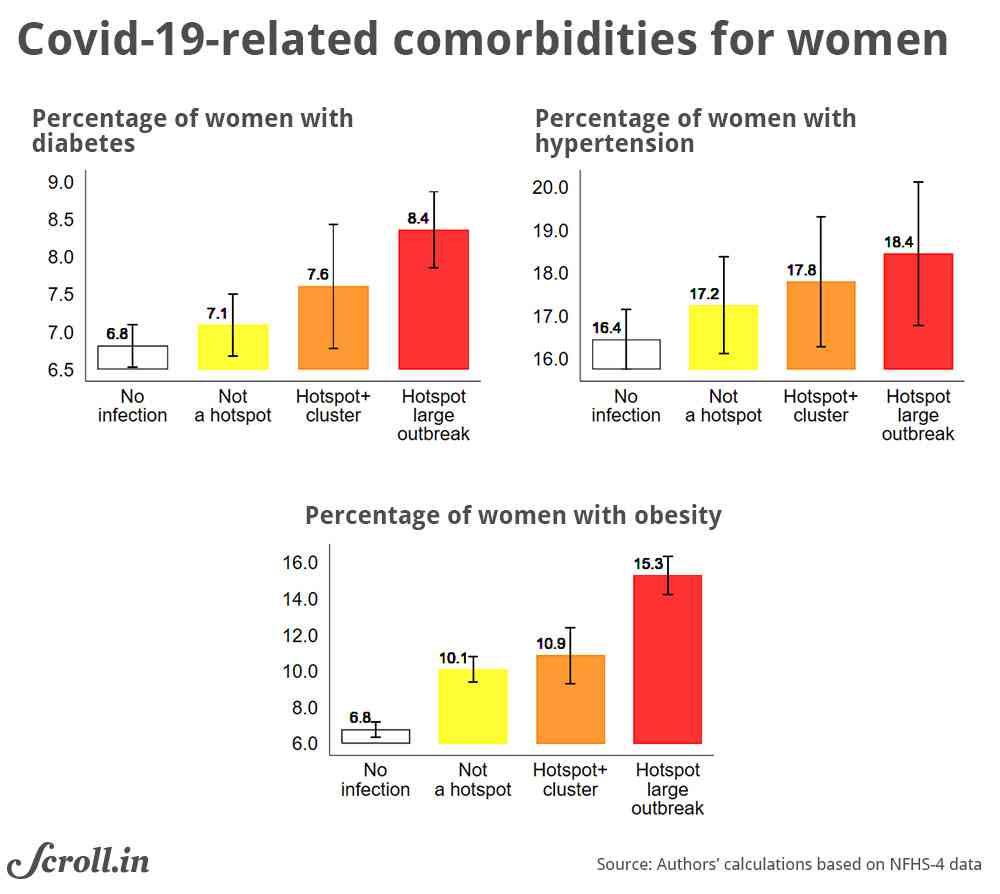
Research has documented that Covid-19 risk factors are associated with a long-term exposure to fine particulate matter and smoking. Indeed, there is a strong empirical link between exposure to air pollution and Covid-related mortality in the United States and Italy. In India’s case, several hotspot districts did have a higher concentration of fine particulate matter in air (PM2.5). But this relation does not persist outside if we exclude Delhi, Haryana or Punjab, which have many hotspot districts.
On a positive note, tobacco consumption in hotspot districts is lower, and this is unlikely to be a major risk factor, at least now.
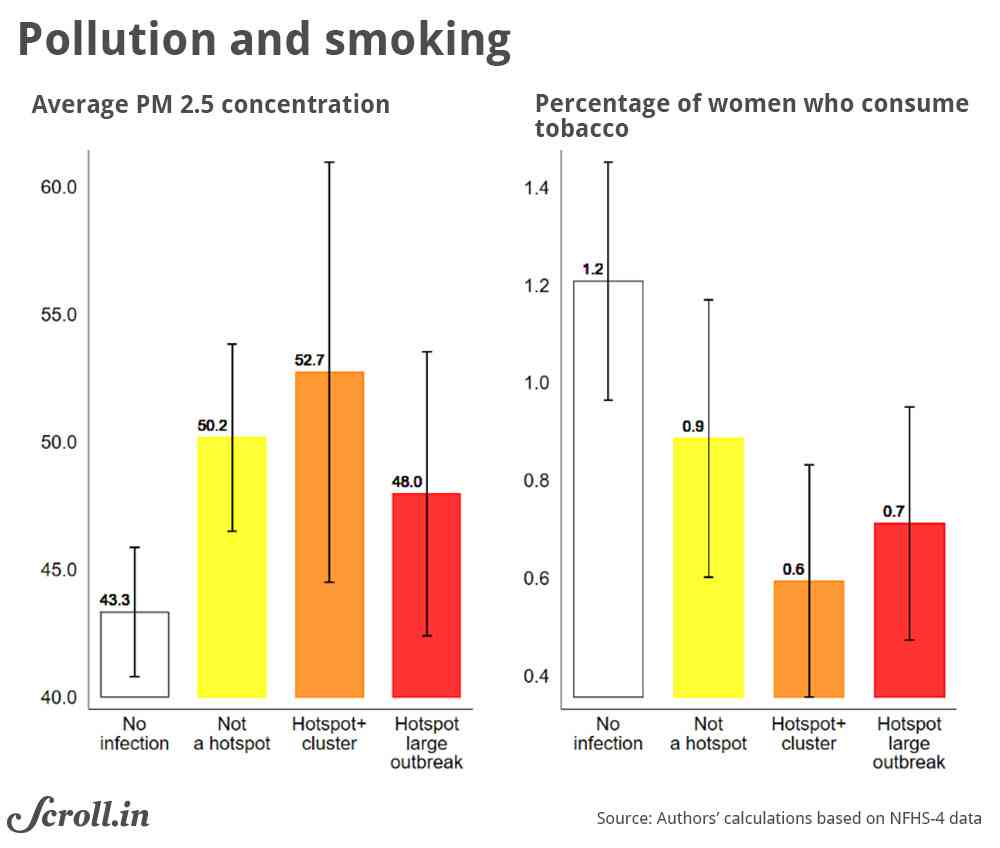
To summarise, we find that the hotspot districts are more prosperous, denser, and urban, where containing the spread may be more challenging. But there is still a considerable population of poor people in these districts who may not be able to access government social protection. The burden would also be more intense for women during social distancing due to limited access to water.
Descriptive patterns show that current hotspot districts bear a large burden of comorbidities, and protecting districts with large at-risk populations would be especially critical. It is worth reiterating that various features of hotspot districts are typically coincidental and do not predict future outbreaks.
The battle against Covid-19 will be a long one, and it will be a while before a vaccine is available at scale. As the social distancing restrictions are eased in currently unaffected districts, it is vital to have a wide social safety web in place because they have a higher concentration of poor populations.
As India grapples with the pandemic and its consequences, it will be imperative to use all kinds of data to understand the socio-economic and risk landscape in both currently affected and unaffected districts. This exercise is an attempt to use publicly available geocoded datasets to this end.
Madhulika Khanna and Nishtha Kochhar are PhD candidates at the Department of Economics, Georgetown University.